
교사의 생성형 AI 수용 모델 프레임워크 개발
Copyright ⓒ 2025 The Digital Contents Society
This is an Open Access article distributed under the terms of the Creative Commons Attribution Non-CommercialLicense(http://creativecommons.org/licenses/by-nc/3.0/) which permits unrestricted non-commercial use, distribution, and reproduction in any medium, provided the original work is properly cited.
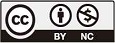
초록
본 연구는 교사의 생성형 AI 사용 의도를 설명하기 위한 프레임워크를 개발하고 검증하는 데 목적이 있다. 이를 위해 기술 수용 모델(TAM), 통합 기술 수용 이론(UTAUT), 가치 기반 수용 모델(VAM), 후기 수용 모델(PAM)에서 도출된 주요 변수들을 교사 중심으로 재정의하고, 교육적 맥락에 맞게 수정하여 사용하였다. 연구 결과, 교육 성과 기대, 사용 편의성 기대, 즐거움, 비용-혜택 인식, 기대 일치, 동료 및 조직의 지원, 습관 변수가 독립변수로 선정되었으며, 나이, 전공, 교직 경력이 조절 변수로 검증되었다. 특히, 습관과 사용 편의성 기대 변수가 가장 중요한 요소로 평가되었으며 이러한 모델은 교사들의 생성형 AI 수용을 촉진하기 위한 교육 프로그램 개발 및 정책 설계에 활용될 수 있다.
Abstract
This study aims to develop and validate a framework to explain teachers' acceptance intentions of generative artificial intelligence (AI). To achieve this, key variables derived from the Technology Acceptance Model (TAM), Unified Theory of Acceptance and Use of Technology (UTAUT), Value-Based Adoption Model (VAM), and Post-Acceptance Model (PAM) were redefined and adapted to the educational context, with a focus on teachers. The results identified educational performance expectancy, ease of use expectancy, enjoyment, cost-benefit perception, expectation confirmation, peer and organizational support, and habit as independent variables, along with the validation of age, major, and teaching experience as moderating variables. As a result of the research, habit and ease of use expectancy were evaluated as the most critical factors. This model framework can serve as a foundation for designing educational programs and policies to promote teachers’ acceptance of generative AI.
Keywords:
Generative AI, Technology Acceptance Model, United Theory of Acceptance and Use of Technology, Value Acceptance Model, Post-Acceptance Model키워드:
생성형 AI, 기술 수용 모델, 통합 기술 수용 이론, 가치 기반 수용 모델, 후기 수용 모델Ⅰ. 서 론
디지털 대전환 시대를 맞이하게 되자 인공지능은 점점 더 빠른 속도로 다양하게 발전하면서 사람들의 생활 방식이나 패턴에 큰 영향을 미치고 있으며 산업 전반에 중요한 역할을 하고 있다[1]. 특히, 사용자와 상호작용을 통해 답변을 제시하기도 하고 학습하면서 새로운 결과물을 만들어내는 생성형 AI가 단연 독보적인 존재로 주목받고 있다[2]. 이런 특성으로 인해 학생들의 눈높이를 고려한 맞춤형 학습 콘텐츠를 제공해 줄 수 있다는 기대감에 교육 분야에서도 많은 연구가 진행되고 있다[3].
그런데 교육 현장은 생성형 AI 도입이 초기 단계인 만큼 생성형 AI 사용 지침이나 정책에서 혼선이 있다. 비단 이런 문제뿐만 아니라 교사의 생성형 AI 사용 의도에 따라서도 교육의 질적 차이가 나타나고 있다. 새로운 기술을 받아들이는데 적극적인 교사는 새로운 기술을 도입하여 학생들의 학습 능력을 향상하고자 노력한다. 하지만 기술을 소극적으로 수용하는 교사는 새로운 기술을 활용하여 학생들을 가르치는 것에 대해 거부감이 있다[3].
이런 상황에서 새로운 기술이 도입되었을 때 사용자가 어떻게 받아들이고 사용 의도를 파악하기 위해 기술 수용 모델, 통합 기술 수용 이론, 가치 기반 수용 모델, 후기 수용 모델을 이용하여 생성형 AI 사용 의도를 파악하는 연구가 진행되고 있다. 하지만 이런 연구의 초점이 교사가 아닌 일반적인 사용자 위주이다. 그러므로 교사라는 직업군의 특성을 반영하여 기존 기술 수용 이론에서 사용된 변수를 재정의해야 한다. 또한 특정 모델이나 이론 중심으로 사용 의도를 분석하기 때문에 다양한 모델에서 이용된 변수의 특성 및 그 모델이 제안된 배경 및 이유를 살리지 못해 사용 의도를 체계적으로 고찰하고 파악하는 데 어려움이 있다.
따라서 본 연구는 기존 연구를 바탕으로 다양한 새로운 기술 중에서 생성형 AI를 새로운 기술로 한정하고 사용자를 교사로 제한하고자 한다. 또한 사용 의도를 파악하는 다양한 모델에서 이용된 변수들을 분석한 뒤 교사의 생성형 AI 사용 의도를 체계적으로 측정할 수 있는 프레임워크를 만들어 생성형 AI 도입에 따른 교육 현장의 혼선을 줄이고자 한다.
Ⅱ. 이론적 배경
본 연구에 있어서 중요한 개념인 생성형 AI와 기존 선행 연구로 제시된 다양한 기술 수용 이론 및 생성형 AI 사용 의도에 관한 연구를 정리하고자 한다.
2-1 생성형 AI
생성형 AI는 AI의 한 분야로 빅데이터를 바탕으로 입력된 데이터를 학습하여 새로운 콘텐츠를 창조해 내는 기술로 정의할 수 있다[4]. 그래서 인간의 언어를 학습하여 문장, 이미지, 음성, 비디오 등을 자동으로 생성하고 있다[5]. 특히 교육 분야에서 생성형 AI는 학생 맞춤형 답변을 제공하여 학습 동기가 부족한 학생들에게 개별화 학습을 지원해 줄 수 있어 심리적 좌절감을 낮추는 데 도움이 된다[6]. 그러나 생성형 AI가 만들어낸 콘텐츠는 데이터를 학습한 결과물이므로 정확하지 않거나 신뢰성이 떨어질 수 있으며 저작권 문제가 발생할 수 있다. 그래서 생성형 AI를 사용할 때는 항상 윤리적 문제를 고민해야 한다[7].
2-2 기술 수용 이론의 개관
기술 수용 모델은 합리적 행위 이론에 기반하여 새로운 기술이 도입하였을 때 그 기술을 수용하는 사람의 사용 의도를 설명하기 위한 모델로 Davis가 처음 제안했다. 이 이론에는 ‘인지된 유용성’과 ‘인지된 용이성’이라는 두 개의 중요한 변수가 사용된다. 이 두 개의 핵심 변인을 바탕으로 사용자가 새로운 기술을 쉽게 이용하여 업무의 효율을 개선할 수 있다고 느껴지면 실제 사용으로까지 큰 영향을 미친다는 점을 설명하고 있다[8]. 그러나 기술 수용 모델은 여러 한계점이 있어 상황에 따라서 학자들에 의해 다양하게 변형 및 발전되어 나갔다.
통합 기술 수용 이론은 기술 수용 모델이 간소화되어 있다는 점을 보완하기 위해 복잡한 정보 기술의 상호 관계를 정확히 분석하고자 기술 수용과 연관이 있는 8개의 이론 및 32개의 개념을 통합한 이론이다. 통합 기술 수용 이론이 도입된 초기에는 조직 내에서 정보 기술 수용 과정을 설명해 준다고 인정을 받았지만, 어느 정도 제한점이 있었다[9]. 그래서 일반적인 소비자 행동의 특성을 설명하기 위해 변수로 쾌락적 동기, 가격 가치, 습관을 추가하고 조절 변수로 성별, 나이, 경험으로 하는 통합 기술 수용 이론 2를 제안했다[10].
가치 기반 수용 모델은 기술 수용 모델이 기술의 수용 과정에서 발생하는 긍정적 요인만 고려되었다는 비판에서 제안되었다. 가치 기반 수용 모델은 기술을 받아들이는 과정에서 사용자가 새로운 기술 수용을 통해 받는 혜택과 희생을 종합적으로 고려하여 가치를 평가한 뒤, 가치가 극대화가 될 때 기술 수용이 합리적이라고 판단하는 모델이다[11].
후기 수용 모델은 기술 수용 모델이 정보 기술을 사용하려는 사용자의 의도에만 초점을 맞추고 있다. 그래서 기술을 수용한 뒤 지속해서 사용할지에 대한 설명이 부족하다는 점에서 제안되었다. 따라서 후기 수용 모델은 기술 수용 모델과 수용 전 기대와 실제 사용한 뒤 어느 정도 충족이 되었는지 설명하는 기대 일치 이론을 근간으로 지속적 사용 의도를 파악하고자 하는 모델이다[12].
이러한 모델의 특징을 정리하면 표 1과 같이 정리할 수 있다. 또한 각 모델에서 사용된 독립변수에서 교사의 생성형 AI 수용 모델에 이용될 변수를 선별하기 위해 비슷한 것끼리 묶으면 표 2와 같이 나타낼 수 있다.
2-3 교사의 생성형 AI 사용 의도
이보영은 교사의 생성형 AI 사용 의도를 파악하기 위해 기술 수용 모델에 디지털 수업 몰입이라는 매개 변인을 추가해서 분석했다. 연구 결과 교사의 몰입이 생성형 AI 사용 의도를 향상하는 데 중요한 경로임을 입증하였지만, 나이와 성별 및 경험과 같은 개인적 특성을 고려한 분석이 이루어지지 않았다[3].
민보경은은 통합 기술 수용 이론을 바탕으로 챗GPT 및 생성형 AI 서비스 이용에 영향을 미치는 요인을 연구했고 조절 변수로 나이를 도입했다. 통합 기술 수용 이론에 사용된 독립 변수가 모두 사용 의도에 유의미한 결과를 도출했으며 연령의 조절 효과는 부분적으로 채택되었다. 그러나 통합 기술 수용 이론의 조절 변수로 나이만 고려되었을 뿐 다른 조절 변수에 관한 연구는 이루어지지 않았으며 표본 대상이 교사가 아닌 다양한 직군으로 이루어져 있다[13].
선덕길은 가치 기반 수용 모델을 기반으로 가치와 만족이 매개 변수로 작용하여 생성형 AI 서비스 지속 사용 의도에 미치는 영향을 분석했다. 하지만 생성형 AI 서비스를 ChatGPT에 한정하여 지속 사용 의도를 연구했으며 사용자의 종사하는 분야별 특성을 고려하지 않았다는 제한점이 있다[14].
차나래는 MICE산업 종사자 대상으로 생성형 AI 지속 사용의도에 미치는 영향을 연구하기 위해 생성형 AI 사용 특성과 후기 수용 모델의 인지된 유용성과 기대 일치를 매개 변수로 사용했다. 하지만 MICE 산업의 세부적인 영역까지 다루지 못했다는 한계가 있다[12].
사용 의도에 관한 선행 연구를 종합하자면 특정 직군 대상으로 한 연구가 미비하거나 변수를 좀 더 세분화, 다양화할 필요성을 언급하고 있다. 따라서 본 연구는 사용 의도를 파악할 수 있는 다양한 이론들을 종합한 뒤 교사에 제한을 두어 연구하고자 한다.
Ⅲ. 교사의 생성형 AI 수용 모델 프레임워크 정의 및 구성 요소
3-1 프레임워크 개발 방향
본 연구에서는 사용자들이 새로운 기술을 받아들일 때 사용 의도를 알아보는 선행 연구를 검토하여 생성형 AI 및 교사의 특성이 반영된 교사의 생성형 AI 수용 모델 프레임워크를 제안하고자 다음과 같은 사항을 고려했다. 첫째, 기술 수용과 관련된 이론에서 많이 언급되는 주요 변수의 가중치를 높게 두고자 한다. 표 2에서 모형별로 변수들을 비슷한 것끼리 묶어서 정리한 뒤 가장 많이 언급되는 변수인 인지된 유용성과 인지된 사용 용이성에 가장 높은 가중치를 둔다. 다음으로 즐거움과 가격 가치를 두 번째로 높은 가중치로 설정하고 나머지 변수를 가장 낮은 가중치를 두어 설정한다. 둘째, 생성형 AI의 특성을 반영하여 나머지 변수 중에서 기대 일치 및 만족을 묶어서 하나의 변수로 설정하고 즐거움, 가격 가치와 함께 두 번째로 중요한 변수로 가중치를 주고자 한다. 생성형 AI는 프롬프트를 입력하는 과정에서 거짓을 마치 사실처럼 말하는 Hallucination 현상이 발생한다. 이 현상은 후기 수용 모델에서 사용하기 전과 사용한 후에 이용자의 지속적 사용 의도를 설명하기 위한 만족, 기대 일치 변수와 비슷한 맥락에서 중요하다. 셋째, 교사는 학생들을 가르치고 학교에 소속되어 교육과 관련된 행정 업무를 한다. 그러므로 교사의 생성형 AI 수용 모델 프레임워크의 변수를 정의할 때 이러한 측면을 반영하여 기존에 기술 수용과 관련된 모델에서 교육 및 업무의 관점에서 변수를 새롭게 정의하고자 한다. 아울러 통합 기술 수용 이론에 이용된 조절 변수를 참고하여 교사의 프로파일링을 성별, 나이, 전공, 교직 경력으로 나누고 조절 변수로 설정했다. 표 3은 교사의 생성형 AI 수용 모델 프레임워크에 사용될 독립변수이고 그림 1은 변수별로 가중치를 두고 도식화하여 나타낸 것이다.
3-2 연구 방법
교사의 생성형 AI 수용 모델 프레임워크를 개발하기 위해 1차로 기초적인 문헌 연구 작업을 했다. 이렇게 문헌 연구를 통해 나온 결과를 바탕으로, 2차로 연구진이 모여 본 연구의 목적과 개발 방향에 맞게 변수가 제대로 정의되었는지, 가중치가 적절한지 등을 논의하여 초안을 그림 1과 같이 작성했다. 그리고 이에 대한 타당도 검증을 위해 12명의 전문가에게 변수에 대한 타당도 및 가중치의 적절한지 물어보았다.
우선, 변수의 타당도를 측정하기 위해 5점 리커트 척도를 문항 구성한 뒤, Lawshe의 내용 타당도 비율(CVR, Content Validity Ratio) 계산식을 이용하고자 한다. 내용 타당도 비율은 어떤 문항이 내용을 적절하게 측정한다고 응답한 비율을 선형적으로 나타낸 값을 의미한다[15]. 패널수에 따른 최소 CVR 값을 표 4와 같다[16]. 본 연구에서 12명의 전문가 응답을 받았기 때문에 CVR의 최솟값을 0.56으로 설정했다.
독립변수의 가중치가 적절한지 알아보기 위해 의사 결정 계층 분석(AHP) 기법을 이용했다. 이 기법은 Thomas L. Satty 교수가 여러 가지 변수들을 쌍대 비교하여 중요성을 판별하기 위해 개발한 기법이다[17]. 쌍대 비교는 여러 요소의 우선순위를 파악하기 위해 모두 함께 평가하는 것이 아니라 두 요소만 정량적으로 비교한 뒤 그 결과를 최종적으로 정리한다. 이렇게 하면 의사결정자의 심리적 부담감을 덜어내 수 있다[18]. 또한, 설문 응답자들로부터 AHP 분석 기법을 적용하여 다양한 판단을 수치상으로 통합하여 우선순위를 도출할 수 있다[19]. 본 연구에서 이용된 독립변수는 7개이므로 최대 고유값(λ-max)이 7에 가까울수록, 일관성 지수(Consistency Index, CI)가 0에 가까울수록, 일관성 비율(Consistency Ratio, CR)이 0.1 미만으로 나왔을 때 변수의 우선순위가 신뢰한다고 볼 수 있다.
타당도 검증 및 가중치를 설정하기 위해 조사에 참여한 12명의 전문가는 컴퓨터 및 인공지능 교육 관련 석사 학위 이상의 교육전문직 1명, 초등 교사 9명, 중등 교사 2명으로 포함되어 있다. 또한 실제 교육 현장에서 SW·AI 교육을 운영해 본 사람으로 구성했다.
Ⅲ. 연구 결과
4-1 타당도 검증 결과
전문가들이 생각하는 교사의 생성형 AI 수용 모델 프레임워크 초안에 제시된 독립변수에 대한 타당도는 표 6과 같이 나타났다. 모든 독립변수에 대한 타당도가 0.56 이상으로 교사의 생성형 AI 수용 모델 프레임워크에 독립변수로 사용하는데 적절하다는 결론을 도출했다.
조절 변수의 타당도는 표 7과 같이 성별을 제외한 나머지 조절 변수는 CVR이 모두 0.56 이상으로 타당도가 검증되었고 성별이 적절하지 않은 것에 대한 추가 심층 질문을 했다. 그 결과, 성별을 조절 변수로 설정하는 것은 남성이 기술 친화적이고 여성은 그렇지 않다는 본질주의적 성별 고정관념을 강화할 수 있다는 점과 다른 조절 변수에 비해 성별 차이가 기술 수용에 미치는 영향과 관련성이 미비하다는 의견을 받았다.
4-2 가중치 순위 결과
7개의 독립변수를 쌍대 비교를 통한 AHP 분석 기법을 한 결과 λ-max는 7.023, CI는 0.003, C.Ratio는 0.003이 나왔다. 신뢰할 수 있는 수치이므로 조사 결과는 믿을만한 자료로 채택되었고 항목별 순위는 표 8과 같이 도출되었다. 습관 변수가 제일 높게 나온 이유는 생성형 AI 이용 빈도가 높을수록 사용 의도가 커진다는 것으로 해석된다. 이는 생성형 AI가 학교 현장에 많이 도입되었으나 사용하는 사람만 주로 이용하므로 기존의 방식을 탈피할 수 있는 지원이 필요하다는 점을 알 수 있다. 또한, 사용 편의성 기대 변수가 두 번째로 높게 나온 이유는 생성형 AI에 대한 접근성 및 이용 방법에 진입 장벽이 낮아야 쉽게 수용할 수 있음을 의미한다. 이는 생성형 AI를 활용하여 수업 준비와 교육 활동에서 얼마나 쉽게 사용할 수 있는지 실질적으로 느낄 수 있어야 한다는 것이다.
4-3 교사의 생성형 AI 수용 모델 프레임워크 도식도
전문가들의 의견을 수렴하고 반영한 뒤 교사의 생성형 AI 수용 모델 프레임워크를 최종적으로 그림 2와 같이 제안한다. 반영된 의견은 다음과 같다. 첫째, 기존에 설정한 독립변수와 정의는 모두 타당도를 입증받았기 때문에 그대로 두었다. 다만, 조절 변수에서 타당도를 입증받지 못한 성별을 제외하여 신뢰성을 향상했다. 둘째, 독립변수 간에 항목별 가중치를 설정했다. 기존 생성형 AI 수용 모델 프레임워크 초안에는 독립변수의 가중치를 3단계로 표현했다. 이에 따라 변수별 가중치를 정확하게 표현하지 못하는 단점이 있다. 이를 해결하기 위해 AHP 분석 기법 결과를 고려하여 퍼센트(%)로 비율을 미세하게 조정했다. 습관(18%), 사용 편의성 기대(16.3%), 교육 성과 기대(14.3%), 즐거움(13.5%), 비용 혜택 인식(12.9%), 기대 일치(12.6%), 동료 및 조직의 지원(12.4%)으로 두어 1순위부터 7순위까지 항목별로 소수점 첫째 자리까지 표현하여 프레임워크의 정확성을 높였다.
Ⅳ. 결론 및 제언
4-1 결론
본 연구는 교사가 나날이 발전해 가는 생성형 AI를 수용할 때 미치는 요인을 관련 연구 및 문헌 분석을 통해 변수를 새롭게 정의하고 가중치를 설정하여 교사의 생성형 AI 수용 모델 프레임워크를 제안했다. 전문가들로부터 타당도 조사를 통해 7개의 독립변수, 3개의 조절 변수를 검증받았다. 또한 AHP 분석 기법을 통해 7개의 독립변수를 계층화하여 각 변수별 가중치를 도출했다.
본 연구 결과를 통해 교사 대상의 생성형 AI 사용 의도를 체계적으로 파악할 수 있는 프레임워크를 제시했다는 점에서 의의가 있다. 생성형 AI를 적용하여 교육 프로그램을 개발하고자 하는 교사의 연수를 계획하고 운영하는 데 도움이 될 것이다. 또한 교사 본인의 생성형 AI 사용 의도를 스스로 점검하여 생성형 AI를 교육에 잘 적용할 수 있을지 파악하고 보강할 수 있는 방향성을 제시할 수 있을 것이다.
4-2 제언
본 연구는 교사의 생성형 AI 사용 의도를 파악하는 프레임워크를 제안했다. 그러므로 향후 연구로 다음과 같이 제시할 수 있다.
첫째, 프레임워크를 바탕으로 생성형 AI 사용 의도를 측정 및 진단해 보아야 한다. 실제 교사들의 생성형 AI 사용 의도를 조사하고 측정 데이터를 기록 및 정리하여 교육 분야별로 상세한 수준으로 프레임워크를 보완하면서 개발해야 한다. 특히 본 연구는 교사의 생성형 AI 수용 모델 프레임워크 개발 초기 단계이므로 교사의 내적 요인에 초점을 두었다. 그러므로 학교 환경과 같은 외적 요인을 반영하여 정교하게 교사의 생성형 AI 사용 의도를 파악할 필요성이 있다. 또한, 생성형 AI 사용 의도가 낮은 교사부터 높은 교사별로 맞춤형 교육 프로그램을 제시해야 한다. 이런 연구를 통해 교육 현장에 생성형 AI 도입을 자연스레 이끌어낼 수 있다. 특히 습관 및 사용 편의성 기대를 올릴 수 있도록 교사 대상으로 내실있는 생성형 AI 활용 연수와 같은 교육적 지원 및 행정적으로는 구독료 지원을 위한 교사용 계정 발급 또는 절차를 간소화할 필요가 있다.
둘째, 학생 대상으로 생성형 AI 사용 의도를 파악할 수 있는 연구를 해야 한다. 본 연구에서 사용 의도를 정확히 측정하기 위해 연구 대상을 교사로 제한했다. 그러나 학생 또한 생성형 AI 사용 의도에 따라 생성형 AI 도입에 대한 거부감이 다를 것이다. 그런데 교사와 학생은 인지적, 정의적, 행동적 요소가 다르다. 따라서 학생의 생성형 AI 사용 의도를 제대로 파악하기 위해 학생 집단으로 한 또 다른 생성형 AI 수용 모델 프레임워크를 연구하여 제시할 필요가 있다.
References
-
S.-Y. Choi, “A Study on the AI Literacy Framework,” The Journal of Korean Association of Computer Education, Vol. 25, No. 5, pp. 73-84, September 2022.
[https://doi.org/10.32431/kace.2022.25.5.007]
-
J. Lee, Determinants of Continuous Use of Generative AI: Focusing on the Extended Technology Acceptance Model (ETAM) and AI Literacy, Master’s Thesis, Hanyang University, Seoul, February 2024.
[https://doi.org/10.16914/kjapr.2024.26.3.96]
-
B. Lee, Analysis of Factors Affecting Teachers’ Intention to Use Generative AI: Focused on the Technology Readiness Acceptance Model, Ph.D. Dissertation, Chung-Ang University, Seoul, February 2024.
[https://doi.org/10.23169/cau.000000241753.11052.0000574]
-
J. V. Pavlik, “Collaborating with ChatGPT: Considering the Implications of Generative Artificial Intelligence for Journalism and Media Education,” Journalism & Mass Communication Educator, Vol. 78, No. 1, pp. 84-93, March 2023.
[https://doi.org/10.1177/10776958221149577]
-
S. I. Yun, A Study on the Impact on Behavioral Intention Generative AI(GenAI) Services -Focusing on UTAUT2-, Ph.D. Dissertation, Dongguk University, Seoul, February 2024.
[https://doi.org/10.23216/dgu.000000087996.11020.0000103]
- J. K. Lee, The Impact of a Python Project Class Using Generative AI as an Individualized Learning Feedback Tool on High School Student’s Self-Efficacy and Computational Thinking: With a Focus on Using ChatGPT, Master’s Thesis, Korea National University of Education, Cheongju, February 2024.
- J. S. Jo, Study on the Improvement of Reading Journal Writing Ability Using Generative AI, Master’s Thesis, Seoul National University of Education, Seoul, February 2024.
- J. Ching, A Study on the Acceptance Intention of Chinese Middle and High School Teachers for Robot Based Education -Based on the TAM(Technology Acceptance Model)-, Ph.D. Dissertation, Wonkwang University, Iksan, February 2019.
-
G. E. Lee, A Study on Factors Influencing User Acceptance Intention of Service Robots, Ph.D. Dissertation, Korea University, Seoul, August 2023.
[https://doi.org/10.23186/korea.000000277112.11009.0000124]
- W. S. Cho, A Study on Factors Affecting Usage Intention of Metaverse Services in the Work Environment -An Application of UTAUT2 Model-, Ph.D. Dissertation, Kookmin University, Seoul, February 2023.
-
T. Koo, Analyzing Factors Affecting Intention to Use a Professional Sports Metaverse Platform Using Value-Based Adoption Model (VAM): Focusing on the Comparison between Generation X, and Generation MZ, Ph.D. Dissertation, Chung-Ang University, Seoul, August 2023.
[https://doi.org/10.23169/cau.000000239733.11052.0000530]
- N. R. Cha, The Impact of Generative AI Usage Characteristics on Work Efficiency and Continuance Intention of MICE Industry Workers: Focusing on the Post Acceptanace Model, Master’s Thesis, Kyung Hee University, Seoul, August 2024.
-
B.-K. Min and C-S. Lee, “A Study on Factors Affecting the Use of ChatGPT and Generative AI Services Based on UTAUT Model,” Journal of Next-Generation Convergence Technology Association, Vol. 7, No. 12, pp. 2298-2311, December 2023.
[https://doi.org/10.33097/JNCTA.2023.07.12.2298]
-
D.-K. Seon, A Study on the Factors Influencing User’s Intention to Continue Using Generative AI Services: Focusing on the Value-Based Adoption Model(VAM), Master’s Thesis, Korea University, Seoul, August 2024.
[https://doi.org/10.23186/korea.000000288900.11009.0001568]
-
J. Song, “Development and Validation of Artificial Intelligence Education on the Environmental Education Based on Unplugged,” Journal of the Korean Association of Information Education, Vol. 25, No. 5, pp. 847-857, October 2021.
[https://doi.org/10.14352/jkaie.2021.25.5.847]
-
K. Chung, S. Kim, and H. Kim, “Development of Artificial Intelligence Literacy Framework for General Teachers,” The Journal of Korean Association of Computer Education, Vol. 26, No. 6, pp. 1-14, November 2023.
[https://doi.org/10.32431/kace.2023.26.6.001]
-
T. L. Satty, “Decision Making with the Analytic Hierarchy Process,” International Journal of Services Sciences, Vol. 1, No. 1, pp. 83-98, March 2008.
[https://doi.org/10.1504/IJSSCI.2008.017590]
- H. M. Jeong, The Exploration of the Assessment Standards for Teaching Competency of Specialized High School Teachers by Using Modified Delphi & AHP, Master’s Thesis, Korea National University of Education, Cheongju, February 2020.
- J. O. Ryu, Developing Evaluation Index of Korean Language Education Communicative Competency -Using the Delphi method and Analytic Hierarchy Process-, Ph.D. Dissertation, Kyungnam University, Changwon, February 2021.
저자소개
2013년:제주대학교 교육대학 초등영어교육과 (학사)
2023년:제주대학교 교육대학원 인공지능융합교육전공 (석사)
2023년~현 재: 아라초등학교 교사
2023년~현 재: 제주대학교 일반대학원 과학교육학부 컴퓨터교육과 (박사과정)
※관심분야:생성형 AI, 인공지능융합교육
1989년:서울대학교 컴퓨터공학과(공학사)
1991년:서울대학교 컴퓨터공학과(공학석사)
1995년:서울대학교 컴퓨터공학과(공학박사)
1996년~현 재: 제주대학교 컴퓨터교육과 교수
2020년~현 재: 제주대학교 교육대학원 인공지능융합교육전공 교수
※관심분야:컴퓨팅시스템, SW·AI 교육, 컴퓨터교육 등