
웹툰 제작 과정에서의 인공지능 기술 활용 방안 연구
Copyright ⓒ 2024 The Digital Contents Society
This is an Open Access article distributed under the terms of the Creative Commons Attribution Non-CommercialLicense(http://creativecommons.org/licenses/by-nc/3.0/) which permits unrestricted non-commercial use, distribution, and reproduction in any medium, provided the original work is properly cited.
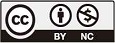
초록
웹툰 시장이 빠르게 성장하면서 웹툰을 서비스하는 다양한 플랫폼이 생겼으며 이에 따라 연재되는 작품들도 폭발적으로 증가하였다. 이러한 상황으로 인해 웹툰 작가들은 상당히 강도 높은 노동을 하고 있으며 이를 개선할 수 있는 효과적인 해결책이 필요한 실정이다. 이에 본 연구에서는 웹툰 제작 과정에 대한 세부적인 분석과 실 종사자들의 인터뷰를 통해 제작 과정의 일부분이 기술로 대체되는 것이 효과적일 것으로 예측하였다. 이를 바탕으로 웹툰 제작 과정에 적용될 만한 그래픽 관련 AI 기술의 사례들을 조사하였으며, AI 기술의 특징 중 학습과 재현 및 자동화를 해결책에 적용될 만한 장점으로 파악하였다. 이에 따라 AI 기술을 웹툰 제작 과정에 접목하여 노동력을 절감하고 창작에 효율성을 증대하는 새로운 프로세스를 제안하고자 한다.
Abstract
With the rapid growth of the webtoon market, various platforms serving webtoons have been created, and the number of serialized studies has exploded accordingly. Thus, webtoon writers are working very intensely, and an effective solution is required to improve this situation. Therefore, in this study, predicting that replacing part of the production process with technology would have a positive effect, interviews were conducted with actual workers, as well as detailed analyses on the webtoon production process. Cases of graphic-related AI technology that can be applied to the webtoon production process were investigated, identifying learning, reproduction, and automation as advantageous characteristics of AI technology that can be applied to the solution. As a result, we present a new process that reduces labor and increases efficiency in webtoon creation by incorporating AI technology into the production process.
Keywords:
Webtoon, Illustration, Artificial Intelligence Technology, Automation, Reproduction키워드:
웹툰, 일러스트레이션, 인공지능 기술, 자동화, 재현Ⅰ. 서 론
웹툰이란 인터넷의 ‘웹(web)’과 만화를 의미하는 ‘카툰(cartoon)’의 합성어로 PC 및 모바일 기기에 맞추어 제작된 만화이다. 웹에서도 만화를 쉽게 접할 수 있게 되면서 웹툰 시장이 활발해졌으며 네이버나 다음 같은 대형 포털 이외에도 웹툰을 서비스하는 여러 플랫폼이 등장하였다. 웹툰을 서비스하는 플랫폼이 점차 다양해짐에 따라 연재되는 작품들도 폭발적으로 증가하였는데, 이로 인해 작품 간의 경쟁이 심화되고 독자들이 작품에 원하는 퀄리티와 분량에 대한 기대감도 커지게 되었다[1].
현재 웹툰 시장에서 작품의 높은 퀄리티와 많은 분량은 경쟁력을 갖추기 위한 필수적인 요소가 되었다. 웹툰 작가는 보통 일주일에 최소한 1편씩의 작품을 연재한다[2]. 가장 대표적인 예로, 한국에서 가장 큰 웹툰 플랫폼인 네이버 웹툰에 연재하는 작가들의 상당수가 최소 40~80컷 이상의 만화를 일주일에 1~2회 업로드한다. 한국콘텐츠진흥원에서 진행한 '2022년 웹툰 작가 실태조사'에 따르면, 이러한 제작 구조에 따라 이들은 하루 평균 10.5시간과 주 평균 5.8일을 창작에 할애하고 있는 것으로 조사되었다[3]. 또한 웹툰의 창작 활동에서 애로사항을 묻는 문항에는 83.6%의 작가가 ‘작업 및 휴식 시간이 부족하다’ 고 답하였다[4].
이처럼 웹툰 작가들은 상당히 강도 높은 노동을 하고 있으며 이를 개선할 수 있는 효과적인 해결책이 필요한 실정이다. 웹툰은 디지털 이미지 형식의 콘텐츠이기 때문에 AI 기술이 적용되기에 적합한 데이터이다. 또한 모든 제작 과정을 디지털상에서 실행할 수 있으므로 새로운 기술이 도입되기에도 적절하다[5]. 이에 본 연구는 이러한 웹툰의 특징에 초점을 맞추고, AI 기술을 웹툰의 제작 과정에 적용하여 작가들의 노동력을 절감할 수 있는 새로운 웹툰 제작 프로세스를 제안하고자 한다.
Ⅱ. 웹툰 제작 과정
웹툰을 제작하는 방식은 모든 과정을 혼자서 진행하는 개인 창작과 역할을 나누어 진행하는 팀 창작으로 분류할 수 있다. 팀 창작은 내부 스튜디오를 구축해 웹툰을 제작하고 납품하는 에이전시 유형의 CP(Content Provider)도 포함되며 대표적으로 웹툰 기획사인 ‘케나즈’ 가 있다[9].
대부분의 웹툰이 비슷한 제작 과정을 거치지만, 높은 완성도의 작화가 필요한 장르의 작품들은 그림 제작 단계에서 콘티, 스케치, 라인, 밑색, 명암, 후보정, 편집 등의 단계로 세분화된다[10]. 개인 창작은 모든 과정을 메인 작가가 혼자 진행하는 반면, 팀 창작은 각 단계에 특화된 인력이 분업과 협력을 거쳐 한 주에 연재할 웹툰을 제작한다. 이 경우에는 메인 작가를 포함해 최소 3명 이상이 함께 협업한다.
작품의 구상 단계는 주로 메인 작가가 담당하며 메인 작가는 1명인 경우와 글과 그림을 2명의 작가가 분업하여 한 팀을 이루는 경우가 있다. 글 작가가 스토리와 글 콘티 등으로 대략적인 연출을 구상하면, 그림 작가가 각 컷의 연출이 담긴 글 콘티를 바탕으로 그림 콘티 작업을 마친다. 그리고 이에 따라 대략적인 스케치를 한 다음에는 그림 작가와 작가의 스타일을 모방한 어시스턴트가 함께 스케치의 클린업 과정인 라인 작업을 완료한다. 컬러 단계의 경우, 라인으로만 그려진 것들을 빈 공간 없이 정해진 컬러로 꼼꼼히 채우는 밑색 단계와 인체와 배경 및 기타 요소들이 가진 구조적인 특징에 대한 이해를 바탕으로 입체감을 더해주는 명암 단계로 나뉘어 작업 된다.
덧붙여 각 단계는 노동의 종류가 분류되어 협업이 이루어지지만, 유동적으로 생략되거나 다르게 세분화될 수 있다.
Ⅲ. 웹툰 작가 심층 인터뷰
3-1 참여자
연구팀은 웹툰 제작 과정에서 실질적으로 AI 활용할 수 있는 가능성을 알아보기 위해 현재 활동하고 있는 웹툰 작가 8명을 대상으로 심층 인터뷰를 진행하였다. 참여자들은 질문지를 미리 숙지한 후 자유로운 분위기에서 인터뷰에 응하였다.
3-2 평가 방법
인터뷰는 표 3과 같이 웹툰의 제작 환경과 이 과정에서 AI가 활용될 가능성을 파악하기 위한 문항들을 중심으로 진행되었다. 참여자들 대부분이 현재 화제가 되고 있는 이미지 생성 AI를 중심으로 한 지식과 견해를 가지고 있었으므로, 현재 서비스되고 있는 네이버 웹툰의 ‘AI painter’와 같은 AI 기술의 사례들이 추가적으로 설명되었다.
3-3 평가 결과
웹툰 제작 환경에 대한 질문에서 대부분의 참가자는 효율적인 작업을 위해 분업을 해본 경험이 있었으며 웹툰 제작에 최적화된 프로그램을 사용하고 있었다. 하지만 오랜 연재 기간 동안 매주 마감을 위해 긴장감을 놓을 수 없다는 심리적 부담감이 있었다. 또한 제작의 효율성을 증대하기 위해 복수의 프로그램을 사용하는 것에도 비용과 시간적인 측면에서 어려움을 겪는다는 것을 알 수 있었다.
AI에 대한 인식과 활용 가능성 부분에서는 인터뷰 초반 참여자들 모두가 이미지 생성 AI가 창작 영역에 무분별하게 침해하는 것에 대해 우려하고 있었다. 이로 인해 AI 기술의 발전 속도에 대한 두려움을 표하기도 하였으나, 그에 못지않게 현재 웹툰 시장의 노동 강도가 자동화의 도입으로 개선될 수 있다는 부분에서는 과반수가 긍정적인 의견을 내비쳤다.
이는 그동안 AI로 인해 작가의 영역이 축소되는 것에 대한 거부감과 학습 데이터들의 저작권 침해 논란이 야기되어 온 것과는 달리, 명확한 범위의 목표를 가지고 올바른 절차를 거쳐 사용된다면 인공지능 기술이 창작 과정에서도 의미 있는 역할을 할 수 있다는 것으로 판단되었다.
웹툰 제작 과정에서 참가자들은 채색 또는 라인 작업과 같은 반복적인 노동에 극심한 피로감을 느끼고 있었으며, 이러한 부분에서 자동화 시스템의 필요성을 제기하였다. 또한, 웹툰의 서사에는 중요하지 않지만 필수적인 요소인 음식, 건물 같은 물체나 군중과 같은 엑스트라를 쉽게 생성할 수 있다면 작업 과정에 큰 도움이 될 것이라는 의견도 이야기하였다.
더불어, 참가자들은 AI가 글 작가와 그림 작가 및 여러 참여 인력들의 협력을 위한 가이드 작성에 도움이 될 것이라고 예상하였다. 글로만 스토리와 연출을 전달하는 것보다, AI 기술을 활용하면 기획 단계에서 글 작가의 구상을 시각적으로 구현할 수 있기 때문이다. 이를 통해 각기 다른 역할을 맡은 인력들이 정해진 연출을 통일되게 이해하고 협력할 수 있을 것으로 기대된다고 하였다.
Ⅳ. 웹툰 제작에 활용 가능한 AI 모델
4-1 AI 모델 사례 조사
앞선 인터뷰에서 확인한 웹툰 작가들의 고충과 현재 화제가 되고 있는 AI 기술의 흐름을 바탕으로 웹툰 제작 과정에서 활용할만하다고 여겨지는 AI 모델의 사례들을 조사하였다. 본 연구에서는 웹툰을 이미지 유형의 콘텐츠라고 분류하였고, 여러 사례들 중에서도 그래픽과 이미지 생성에 적용되는 모델들을 중심으로 서술하였다. 이 과정에서 연구팀은 주로 이미지 생성에 적용되는 기술인 GAN과 Diffusion 모델, 그리고 또 다른 딥러닝 방식 중 하나인 뉴럴 네트워크(Neural Network) 기술을 원천으로 하는 AI 모델들을 연구 목적에 적합한 사례로 판단하였다. 그리고 두 가지 이상의 기술을 함께 사용하여, 단일 기술을 사용할 때의 단점을 보완한 모델들도 함께 조사한 사례에 포함하였다.
GAN의 경우에는 인물의 얼굴에 특화된 모델로 JoJoGAN, AniGAN, Deep Style Transfer 등이 있다. 또한 채색 과정에는 컬러 포인트를 힌트로 하는 AlacGAN과 텍스트 프롬프트를 힌트로 하는 Tag2Pix, 이미지 학습을 가이드로 하는 Seg2pix가 있으며 인물을 제외한 물체들과 배경 이미지를 생성할 수 있는 Pix2Pix가 있다.
Diffusion 모델의 경우에는 특정한 스타일의 학습과 재현이 가능한 모델을 만들 수 있는 LoRA가 있으며 생성한 이미지에서 특정 부분을 지정하여 수정할 수 있는 SmartBrush가 있다. 특히 최근에는 2022년에 생성형 인공지능 스테이블 디퓨전(stable diffusion)이 출시되고 나서, 이를 쉽게 사용하기 위한 Web UI가 공개되었다. 이것으로 다양한 기능을 추가적으로 활용할 수 있게 되었는데, 인물의 자세를 학습한 이미지대로 생성해 주는 오픈포즈와 부분적인 이미지 수정이 가능한 인페인팅 기능이 대표적인 사례이다.
뉴럴 네트워크(Neural Network)는 이미지 생성에 특화된 기술은 아니지만 효율적인 딥러닝 방식으로 이미지 분야에서도 사용된다. 여기에는 겹쳐진 선을 벡터화하여 깔끔하게 만들어 주는 Sketch Simplification, 특정한 스타일을 가진 라인을 다른 이미지에도 적용시킬 수 있는 SketchPatch, 그리고 지정된 영역에 색을 채워 주는 Petalica paint를 예로 들 수 있다. 특히 Sketch Simplification의 경우 추후 GAN을 적용하는 과정을 한 번 더 거쳐 Stylized Sketch Simplification 모델로 발전하였으며 두 가지 모델을 함께 적용한 만큼 결과물의 질이 향상된 것을 확인할 수 있다.
이 외에도 특정 캐릭터의 배색을 학습하여 다른 캐릭터에 적용시키는 Attention-Aware Anime Line Drawing Colorization과 광원의 색을 설정하여 이미지의 빛 보정에 효과적인 ClipDrop Relight, 영역을 지정해 주면 자동으로 명암을 처리해 주는 SmartShadow, 자동화로 처리된 명암의 퀄리티를 향상시켜주는 Eliminating Gradient Conflict 등의 모델이 있다.
4-2 GAN 기반 모델
GAN(Generative Advarsarial Network)은 생성자와 판별자의 경쟁을 통해 학습하는 이미지 생성모델이다. 생성모델(generator)은 최대한 진짜 같은 데이터를 만들기 위한 학습을 하고, 분류모델(discriminator)은 진짜와 가짜를 판별하기 위한 학습을 하며 이 경쟁 관계를 이용해 이미지를 생성한다[11].
그림 2의 JoJoGAN은 참조된 스타일의 이미지를 학습하고, 입력된 이미지에 학습한 이미지의 스타일을 적용하여 이미지를 생성한다[12].
그림 3의 ‘침착한 생성 모델기’는 깃허브(github) 유저 bryandlee가 네이버 웹툰에서 서비스되었던 이말년 작가의 ‘이말년 시리즈’에서 약 500개의 캐릭터 얼굴 데이터를 추출하여 AI 모델을 학습시켰다. GAN Space는 안경과 같은 액세서리의 여부 및 표정 변화의 정도 등 기본값에서 학습 데이터를 기반으로 결괏값을 추정하여 이미지를 생성한다. 또 다른 방법인 Close-form factorization는 웹 브라우저에서 모델을 테스트할 수 있으며 머리카락, 얼굴의 각도 등을 조정하여 결과물을 보여준다[13].
그림 4의 AlacGAN은 학습 데이터와 사용자가 지정한 컬러를 가지고 의도에 좀 더 가까운 결과물을 얻을 수 있는 조건부 적대 네트워크(Conditional Adversarial Networks) 모델이다[14]. 또한 그림 5의 Tag2Pix는 GAN 기반의 라인 채색 방법으로 흑백 선화와 컬러 태그 정보를 입력하면 컬러링 된 이미지를 생성해 준다[15].
4-3 Diffusion 기반 모델
Diffusion 모델은 본래의 데이터에 노이즈를 더하여 이미지를 뿌옇게 만들고, 이 이미지로부터 노이즈를 다시 점차 줄여가면서 조금씩 복원해 가는 과정을 통해 원래 데이터와 비슷한 이미지를 생성하는 모델이다[16].
그림 6과 같은 LoRA(Low-Rank Adaptation)는 확산 모델인 스테이블 디퓨전(stable diffusion)에 추가적인 학습을 더해 원하는 결과물에 더욱더 가깝게 만들도록 하는 모델로 캐릭터, 스타일, 자세 등 세부적인 요소들을 특화하여 학습시킬 수 있다[17]. LoRA는 기존 생성 AI 모델들에 비해 비교적 적은 데이터로 모델의 빠른 학습을 이끌어 낼 수 있는데, 특히 주목할 만한 점은 창작자가 직접 스스로의 창작물을 학습시킬 수 있다는 것이다[18],[19].
그림 7의 SmartBrush는 주변의 이미지 정보를 참고하여 이미지를 재생성하는 일반적인 인페인팅 방식과 달리 사용자가 입력한 텍스트와 마스크 처리한 영역을 힌트로 하여 원하는 방향에 좀 더 가깝게 이미지를 재생성한다[20].
그림 8과 같이 2023년 Stability AI에서 출시한 Stable Doodle AI는 간략한 낙서를 구체화하고 다양한 스타일을 적용하여 이미지를 생성해 주는 모델이다[21].
4-4 복수 기술 기반 모델
위에서 언급한 GAN과 Diffusion 모델 이외에 함께 사용되는 원천 기술로 뉴럴 네트워크(Neural Network)가 있는데, 이는 인간 뇌의 구조와 기능에 의해 영감을 받은 계산 모델이다[22]. 이 모델은 상호적으로 연결된 뉴런이 입력을 받고 복수의 단계를 처리하여 결과물을 출력한다.
Sketch Simplification은 Convolutional Neural Network 기술을 기반으로, 러프하게 그려진 그림 9(A)와 같은 스케치를 그림 9(B)와 같은 단일 라인으로 단순화시켜 준다. 이 모델에서 발전된 Stylized Sketch Simplification은 입력된 이미지에 Sketch Simplification과 같은 학습 과정을 적용하고 사용자가 원하는 방향에 더 가깝게 결과물이 도출될 수 있도록 GAN 기술을 한 번 더 거친다[23]. 이렇게 도출된 결과물은 위의 그림 9(C)와 같이 Sketch Simplification보다 더 섬세하고 형태감이 확실하다.
그림 10의 ClipDrop-Relight는 Stability.ai에서 출시된 재조명 기술이다[24]. 이 모델의 장점은 광원과 반사광의 방향을 자유롭게 설정하고, 각 광원에 원하는 색을 지정할 수 있다는 것이다. 이것은 컬러와 명암 요소를 조절하여 이미지를 원하는 분위기로 연출하거나 수정하려 할 때 효과적이다[25].
그림 11의 SmartShadow는 사용자가 원하는 영역에 그림자를 생성해 주는 모델이다[26]. 이 모델은 Shadow brush, Shadow boundary brush, Global shadow generator 세 가지의 기능을 사용하여 실시간으로 결과물을 보여준다.
4-5 단계별 AI 모델 배치
표 7은 웹툰 제작 단계에서 다루는 이미지가 어떤 객체인지에 따라 AI 모델을 분류하여 정리한 것이다. 연구팀은 생성되거나 처리되는 이미지를 사람과 물체로 분류하고, 만약 두 요소를 포함한 이미지라면 완성되지 않은 초안인지 모든 과정을 거친 완성된 원고인지에 따라 나누었다. 그리고 표 8을 구성하기 위해 인물 이미지의 경우에는 스케치, 라인, 밑색, 명암의 단계를 적용하여 세부적으로 분류하였다. 각 단계별로 배치된 AI 기술들이 해당 과정을 아주 완벽하게 처리하는 것은 아니지만, 최대한 적절한 AI 모델을 배치하고자 하였다.
초고는 초안으로 제작된 콘티이며, 분업화되어 제작되는 작품의 경우 글 작가가 글 콘티를 작성한다. 이 단계에서는 콘티가 연출하려는 방향이 어떤 이미지인지 구체적인 텍스트로 서술하면서 설명을 돕는 이미지 레퍼런스를 첨부하기도 한다. 스케치를 완성된 이미지로 생성해 주는 Stable Doodle, 특정 스타일을 학습하고 텍스트를 입력해 이미지를 생성하는 LoRa, 가이드 이미지의 인물과 같은 자세로 이미지를 생성해주는 WebUI_openpose는 그림을 잘 그리지 못하는 글 작가들이 그림 작가들과의 소통에 활용할 수 있을 것이다.
물체의 제작에 해당하는 AI 모델은 다른 기술들에 비해 비교적 적은데, 이는 이미 웹툰의 배경 작업에서 스케치업과 같은 3D 프로그램이 활발하게 활용되고 있기 때문이다. 하지만 실사 이미지에 적절한 필터 효과를 거쳐 배경 이미지를 제작하는 경우에는 이미지 해상도를 높이고 다양한 스타일의 이미지로의 변환이 가능한 Pix2Pix가 적절한 효과를 보일 것으로 예상된다.
인물의 경우, 웹툰 제작 과정에서 가장 많이 시간이 소요되는 부분이며 정리된 표에서도 대부분의 AI 모델이 이 분류에 속하였다. 스케치를 포함한 라인 부분에서는 작가의 개성을 학습할 수 있는 AI 모델들을 활용할 수 있고 밑색과 명암 작업을 포함한 채색 단계에서는 자동화에 특화된 모델들을 사용하면 수월할 것이다.
완성 원고는 보정 혹은 수정 단계에 해당한다. 수정 작업에서는 특정 부분만 수정이 가능한 SmartBrush와 같은 모델을 포함한다. 또한 보정 작업에서는 광원의 색을 설정해 주면서 작품의 분위기에 맞게 빛 효과를 내줄 수 있는 ClipDrop Relight와 같은 모델이 포함되었다.
Ⅴ. AI 기술을 적용한 웹툰 파이프라인
본 연구는 앞서 조사한 웹툰에 적용될 수 있는 그래픽 관련 AI 모델의 사례들과 웹툰 작가들의 심층 인터뷰를 기반으로 AI 기술을 활용한 웹툰 제작 파이프라인을 제시하려 한다. 제시하고자 하는 파이프라인은 인공지능 모델의 학습에 있어 타인의 저작물이 아닌 창작자 고유의 데이터를 기반으로 하는 것을 기본 조건으로 한다. 또한 결과물을 창작자가 원하는 방향으로 이끌기 위해 각 과정에서 사람의 개입이 필수적인 준 자동화를 지향한다.
그림 12에 따르면, 웹툰에서 AI 기술의 첫 번째 활용 방안은 협업하는 인력들의 원활한 분업화를 위한 가이드 역할로 제시되고 있다. 그림 12의 ‘Conti with text’부터 ‘Sketch’까지의 단계에서 ‘text to image’ 기능을 통해 글 작가와 그림 작가의 콘티 단계를 더 수월하게 할 수 있다. 이는 글 작가가 연출에 관련된 이미지 레퍼런스를 직접 생성하거나, 러프하게 짜인 이미지를 그림 작가에게 전달하여 스케치 작업을 돕는 것을 의미한다.
다음으로, AI 기술을 활용하여 제작 단계의 일부를 자동화함으로써 단순 반복 작업과 고강도의 노동 환경을 개선할 수 있다. 그림 12에 따르면, Sketch 단계 이후에는 배경과 캐릭터 부분을 나누어 작업하게 된다. 배경은 ‘image to image’ 기능을 활용하여 이미지를 생성하고, 캐릭터는 메인 작가의 스타일을 학습한 고유의 AI 모델을 통해 라인 작업에 소요되는 시간을 단축시킨다.
이후 컬러와 명암 작업에서는 앞서 언급한 AI 모델들처럼 사용자가 지정한 색이나 명암 영역을 힌트로 삼아 결과물을 완성해 나간다. 마지막으로, 여기까지의 결과물을 작업자가 수정 및 편집하여 최종 작업을 완료한다.
VI. 결 론
과거부터 만화 제작은 작가가 대부분의 제작 과정에 직접 참여하여 그림을 그렸기 때문에 상당한 노동력이 요구되었다. 이러한 작가들의 노동 환경은 웹툰 산업이 확장됨에 따라 더 많은 콘텐츠를 요구받으며 점점 열악해졌다. 이와 동시에, 최근 디지털 기기의 성능이 폭발적으로 향상되고 웹에서 다양한 유형의 방대한 데이터가 공유되는 환경이 구축되면서 접근하기 어렵다고 느껴졌던 AI 기술을 누구나 쉽게 사용할 수 있게 되었다. 이에 본 연구는 웹툰 산업에서의 노동 강도 심화 문제에 AI 기술의 장점을 접목하여 새로운 프로세스를 제안하고자 한다. 또한 현재 인공지능이 학습하는 데이터들의 제공이 합법적으로 이루어지고 있는지에 대한 논란에서 벗어나기 위해, 창작자들이 자체적으로 보유한 데이터를 활용할 수 있도록 하는 것을 기본으로 한 방법론을 구상하였다.
본 논문에서는 문헌 연구를 통한 웹툰의 제작 과정이 구체적으로 기술되었다. 다음으로 실제 웹툰 작가들의 인터뷰를 통해 웹툰 제작 과정의 실태와 문제점을 파악하고 AI 기술이 긍정적으로 활용될 수 있는 가능성을 예상하였다. 그리고 현재 공개된 AI 모델들을 조사하여 연구의 목적에 가장 적합한 사례들을 선정하였다. 이를 통해 도출된 결과를 분석하여 AI 기술이 웹툰의 세부적인 제작 과정에 도움을 줄 수 있도록 하는 새로운 프로세스를 제안하였다. 이 프로세스는 분업화를 위한 가이드 제작과 고강도의 노동 과정을 대체하는 것에 초점을 맞췄으며 작가들의 창작 영역을 최대한 보장하고 작업의 효율성을 높이는 것에 목적이 있다.
하지만 현재 AI 기술은 모델들을 학습시키기 위해 적법한 절차를 거치지 않고 무단으로 웹상의 이미지를 사용한다는 비판에 직면하고 있다. 이로 인해 저작권에 대한 논란과 예술 업계 종사자들에게서 강한 거부감을 불러일으켰으며, 개발된 기술의 주 사용 대상자들인 웹툰 작가들과 지망생들에게 AI 기술의 사용을 납득시키지 못하고 있는 것이 현실이다. 또한 배경 제작과 같이 준 자동화가 이루어진 일부 과정의 경우 AI 기술이 이를 대체할 만한 효용성을 지니는지에 대해 검증하고 발전시켜야 하는 과제가 남아 있다.
하여 본 연구팀은 추후 제안한 파이프라인을 토대로 AI 모델들을 활용하여 웹툰을 실제로 제작해 보고, 결과물의 완성도가 현재 서비스되고 있는 웹툰에 견줄 만한지 파악하려 한다. 또한 이를 바탕으로 웹툰 작가들이 AI 기술을 활용한 파이프라인을 빠르게 적용시킬 수 있도록 현실성 있는 방안을 강구할 예정이다.
References
- M. S. Kim,“A Study on the Identity and Labor Process of Webtoon Writers Who Lead the Video Culture,” Journal of Acting & Arts (KOSAS), Vol. 28, No. 4, pp. 61-92, November 2022.
- Y. Lee, H. Kim, J. Min, M. Park, and J. Lee, “Condition Survey on the Working Environment and Physical and Mental Health of Webtoon Writter,” in Proceedings of the 68th Fall Conference of the Korean Society of Occupational and Environmental Medicine, Jeju, pp. 47-48, November 2022.
- The Seoul Institute. Seoul Webtoon Writer Class (Level) [Internet]. Available: http://www.sdi.re.kr/node/65839, .
- Hankookilbo. Shade of K Content Sweeping the World [Internet]. Available: https://m.hankookilbo.com/News/Read/A2024010715270003306, .
- W. Chae and H.-J. Kim, “Sketch-Related AI Technologies for Webtoon-Image Production Process,” Journal of Computing Science and Engineering, Vol. 2021, No, 12, pp. 961-962, December 2021.
- S&T Focus. Why Is Synopsis Important in Webtoons? [Internet]. Available: https://www.sntfocus.co.kr/news/articleView.html?idxno=227, .
- Gobae’s Brunchstory. [1]Webtoon: Theory-(2)Text Conti by Story Writer [Internet]. Available: https://brunch.co.kr/@bshot10/93, .
- S. Cho, The Study of Software Used for Webtoon Background -Focusing on the SketchUp Casestudy-, Master’s Thesis, Sejong University, Seoul, February 2023.
- YTN. Webtoons Also Increase Their Competitiveness through Co-Production Like Dramas [Internet]. Available: https://www.ytn.co.kr/_ln/0106_202102210352407083, .
- M. S. Gil, “Research on Simplification and Harpening of Production Programs for Quality Enhancement of Webtoons,” Journal of Digital Convergence, Vol. 14, No. 12, pp. 389-395, December 2016.
- I. Goodfellow, J. Pouget-Abadie, M. Mirza, B. Xu, D. Warde-Farley, S. Ozair, ... and Y. Bengio, “Generative Adversarial Nets,” in Proceedings of Advances in Neural Information Processing Systems 27 (NIPS 2014), Quebec, Canada, December 2014.
-
M. J. Chong and D. Forsyth, “JoJoGAN: One Shot Face Stylization,” in Proceedings of the 17th European Conference on Computer Vision (ECCV 2022), Tel Aviv, Israel, pp. 128-152, October 2022.
[https://doi.org/10.1007/978-3-031-19787-1_8]
- Bryandlee GitHub. Malnyun_Faces. [Internet]. Available: https://github.com/bryandlee/malnyun_faces, .
-
Y. Ci, X. Ma, Z. Wang, H. Li, and Z. Luo, “User-Guided Deep Anime Line Art Colorization with Conditional Adversarial Networks,” in Proceedings of the 26th ACM International Conference on Multimedia, New York: NY, pp. 1536-1544, October 2018.
[https://doi.org/10.1145/3240508.3240661]
-
H. Kim, H. Y. Jhoo, E. Park, and S. Yoo, “Tag2Pix: Line Art Colorization Using Text Tag with SECat and Changing Loss,” in Proceedings of 2019 IEEE/CVF International Conference on Computer Vision (ICCV), Seoul, pp. 9056-9065, October-November 2019.
[https://doi.org/10.48550/arXiv.1908.05840]
- J. Ho, A. Jain, and P. Abbeel, “Denoising Diffusion Probabilistic Models,” in Proceedings of the 34th International Conference on Neural Information Processing Systems, Vancouver, Canada, pp. 6840-6851, December 2020. https://arxiv.org/abs/2006.11239
- Stable Diffusion Art. What are LoRA Models and How to Use Them in AUTOMATIC1111 [Internet]. Available: https://stable-diffusion-art.com/lora/, .
- GitHub, Stable Diffusion Web UI [Internet]. Available: https://github.com/AUTOMATIC1111/stable-diffusion-webui/, .
- Flat Sun’s Tistory Blog. How to Create a Photo Position using WebUI Openpose [Internet]. Available: https://flatsun.tistory.com/3538, .
-
S. Xie, Z. Zhang, Z. Lin, T. Hinz, and K. Zhang, “SmartBrush: Text and Shape Guided Object Inpainting with Diffusion Mode,” in Proceedings of the IEEE/CVF Conference on Computer Vision and Pattern Recognition (CVPR), Vancouver, Canada, pp. 22428-22437, June 2023.
[https://doi.org/10.48550/arXiv.2212.05034]
- Stability AI. Clipdrop Launches Stable Doodle [Internet]. Available: https://stability.ai/news/clipdrop-launches-stable-doodle, .
-
J. Schmidhuber, “Deep Learning in Neural Networks: An Overview,” Neural Networks, Vol. 61, pp. 85-117, January 2014.
[https://doi.org/10.1016/j.neunet.2014.09.003]
-
Q. Lu, Q. Tao, Y. Zhao, and M, Liu, “Sketch Simplification Based on Conditional Random Field and Least Squares Generative Adversarial Networks,” Neurocomputing, Vol. 316, No. 17, pp. 178-189, November 2018.
[https://doi.org/10.1016/j.neucom.2018.07.065]
- Clipdrop by Jasper. Technicalities Behind Image Relighting [Internet]. Available: https://clipdrop.co/blog/relight-technicalities, .
- Clipdrop by Jasper. Official Website [Internet]. Available: https://clipdrop.co/relight, .
-
L. Zhang, J. Jiang, Y. Ji, and C. Liu, “SmartShadow: Artistic Shadow Drawing Tool for Line Drawings,” in Proceedings of 2021 IEEE/CVF International Conference on Computer Vision (ICCV), Montreal, Canada, pp. 5371-5380, February 2021.
[https://doi.org/10.1109/ICCV48922.2021.00534]
-
P. Isola, J.-Y. Zhu, T. Zhou, and A. A. Efros, “Image-to-Image Translation with Conditional Adversarial Networks,” in Proceedings of 2017 IEEE Conference on Computer Vision and Pattern Recognition (CVPR), Honolulu: HI, pp. 5967-5976, July 2017.
[https://doi.org/10.1109/CVPR.2017.632]
-
N. Fish, L. Perry, A. Bermano, and D. Cohen-Or, “SketchPatch: Sketch Stylization Via Seamless Patch-Level Synthesis,” ACM Transactions on Graphics, Vol. 39, No. 6, pp. 1-14, November 2020.
[https://doi.org/10.1145/3414685.3417816]
- Petalica Paint. Official Website [Internet]. Available: https://petalica.com/index_en.html, .
-
X. Liu, W. Wu, H. Wu, and Z. Wen, “Deep Style Transfer for Line Drawings,” in Proceedings of the 35th AAAI Conference on Artificial Intelligence (AAAI-21), Palo Alto: CA, pp. 353-361, May 2021.
[https://doi.org/10.1609/aaai.v35i1.16111]
-
C. W. Seo and Y. Seo, “Seg2pix: Few Shot Training Line Art Colorization with Segmented Image Data,” Applied Sciences, Vol. 11, No. 4, 1464, February 2021.
[https://doi.org/10.3390/app11041464]
-
Y. Cao, H. Tian, and P. Y. Mok, “Attention-Aware Anime Line Drawing Colorization,” in Proceedings of IEEE International Conference on Multimedia and Expo (ICME), Brisbane, Australia, pp. 1637-1642, July 2023.
[https://doi.org/10.48550/arXiv.2212.10988]
저자소개
2018년:경기대학교 애니메이션 영상학(예술학사)
2023년~현 재: 중앙대학교 첨단영상대학원 예술공학 석사과정
※관심분야:Animation, Comics, Contents Design, Media Art 등
2012년:세종대학교 컴퓨터공학/신문방송학(공학사/문학사)
2020년:중앙대학교 첨단영상대학원 예술공학(영상학석사)
2024년:중앙대학교 첨단영상대학원 예술공학(영상학박사)
2024년~현 재: 동양미래대학교 컴퓨터공학부 조교수
※관심분야:AI, HCI, Web, Media Art 등
1995년:중앙대학교 컴퓨터 공학과(공학사)
1998년:Pratt CGIM ComputerMedia(MFA)
2003년~현 재: 중앙대학교 첨단영상대학원 예술공학 교수
※관심분야:Art&Technology. Procedural Animation 등