
추천 시스템의 편향 및 사용자 만족도 탐구: 체계적 문헌고찰 방법을 중심으로
Copyright ⓒ 2022 The Digital Contents Society
This is an Open Access article distributed under the terms of the Creative Commons Attribution Non-CommercialLicense(http://creativecommons.org/licenses/by-nc/3.0/) which permits unrestricted non-commercial use, distribution, and reproduction in any medium, provided the original work is properly cited.
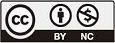
초록
추천 시스템은 사용자 행동에 관한 대량의 데이터를 인공지능으로 학습하여 개인화된 서비스 및 컨텐츠를 제공하는 기술이다. 이러한 기술은 다양한 정보가 혼재된 환경에서 사용자 중심의 초개인화를 가능하게 한다는 점에서 중요하지만, 한편으로는 데이터로부터 주어진 단면만을 학습 및 재강화하여 추천 시스템이 편향될 수 있다는 한계점을 갖는다. 대표적인 추천 시스템의 편향인 필터 버블은 사용자에 맞추어 필터링된 정보만을 활용하게 되는 것을 의미하며, 이는 확증편향과 선택적 인지를 일으키는 부작용을 발생시키며 결론적으로 사용자의 만족도를 하락시킨다. 본 연구에서는 추천 시스템의 편향과 사용자의 만족도 간의 관계를 구체화하기 위하여 국내외 문헌들을 체계적 문헌고찰의 방법을 통해 분석하였다. 일련의 문헌고찰 프로토콜을 활용하여 수집된 문헌을 분석하였으며, 이를 기반으로 (1) 추천 시스템의 편향과 사용자 만족도의 관계, (2) 편향 완화 및 사용자 만족도 향상을 위한 접근법을 분석하였다. 본 연구는 추천 시스템의 새로운 알고리즘이 아닌 편향, 사용자 만족도 등의 새로운 관점에 대한 접근을 수행한다는 점에서 의의를 가진다.
Abstract
A recommendation system aims to provide personalized services or content by learning a huge amount of user behaviors with artificial intelligence. It enables a user-centric personalization in a heterogeneous setting, while the recommendation system can also be biased by focusing on only a limited viewpoint. A filter bubble, i.e., a typical bias of a recommendation system, signifies the system utilizes filtered data according to the user; thus, it causes a side effect of confirmation bias and selective recognition, which in turn leads to a decrease in user satisfaction. In this study, we employed a systematic literature review method to specify the relationship between the bias of the recommendation system and user satisfaction. Based on a series of literature review protocols, we analyzed the articles from three angles: (i) the relationship between the bias of the recommendation system and user satisfaction and (ii) how to mitigate bias and improve user satisfaction. This research contributes to investigating a new angle, i.e., the relationship between bias and user satisfaction, rather than a new technical algorithm of the recommendation system.
Keywords:
Literature Review, Recommender System, Bias, User Satisfaction, Filter Bubble키워드:
문헌고찰, 추천 시스템, 편향, 사용자 만족도, 필터 버블Ⅰ. 서 론
최근 인공지능은 빅데이터의 생성 및 수집, 클라우드 컴퓨팅 및 컴퓨팅 시스템 등 기반 기술의 발달과 함께 급격히 발전하고 있다. 이러한 인공지능 기술은 인간의 고차원적인 정보처리능력을 구현해 나가고 있으며, 이에 따른 다양한 응용 사례를 만들어내고 있다. 그 중 대표적인 응용 분야는 데이터에 기반한 개인화이며, 추천 시스템(Recommendation systems)이라는 기술로 발전되어왔다[1].
추천 시스템은 사용자의 행동을 누적한 데이터를 바탕으로 사용자 개인의 스타일과 특성을 파악하고 이를 통해 다양한 관점에서의 잠재적 선호도를 추출하며, 이를 학습한 결과를 바탕으로 개인별 맞춤 서비스 및 컨텐츠를 제공하는 알고리즘이자 시스템이다[2]. E-commerce, over-the-top media service(OTT) 등 B2C(Business to Customer) 서비스를 제공하는 국내외 다양한 기업에서는 자체적인 추천 시스템 개발 및 구축을 통해 이를 상업적 용도로 활용하고 있다. 주로 개인에게 적합한 특정 아이템을 추천하는 방향으로 적용하고 있으며, 이는 명시적, 암묵적 선호도를 모두 분석하여 반영한다는 특징을 갖는다[3].
다양한 정보가 혼재된 환경에서 사용자 중심의 개인화를 가능하게 하는 추천 시스템의 장점과 더불어, 모수를 그대로 반영한다는 특징으로 인해 추천 시스템이 편향될 수 있다는 한계점도 가진다. 즉, 주어진 단편적인 데이터만을 활용할 경우 현실 세계의 편견을 알고리즘이 학습 및 재강화할 수 있다는 것이다[4]. 이러한 추천 시스템의 편향 문제를 해결하지 않는다면 추천 알고리즘 그 자체만으로 현실 세계의 기존 편향을 강화하거나 더 나아가 새로운 편향 및 불공정 문제를 일으킬 수 있다[5]. 가장 대표적인 추천 시스템의 편향은 ‘필터 버블’ 문제이다. 필터 버블은 점차 사용자 프로필과 유사한 아이템만을 추천하게 되는 특징을 갖는다[6]. 이러한 알고리즘은 평가 방법의 하나인 정확도 측면에서는 높은 수치를 얻을 수 있지만, 사용자에게 적합한 추천인지에 대해서는 의문 부호가 남겨질 수 있다.
추천 시스템의 편향 및 문제점을 해결하기 위해 국내외에서 일부 방법을 제시하였다. 그러나, 대부분 새로운 추천 시스템 알고리즘 개발에 중점을 두고 있으며, 개인화 서비스의 발전이 비교적 최근임에 따라 관련 연구가 많지 않다. 또한, 국내에서는 데이터 보안 관련 법에 따라 사용자의 행동 정보를 활용하는 것이 어려웠으며, 이로 인해 타 국가보다 추천 시스템과 관련한 이슈가 학술적으로 논의되지 못하였다[7].
본 연구에서는 편향 문제를 해결하는 추천 시스템 개발에 앞서, 체계적 문헌고찰 방법을 통해 추천 시스템의 편향에 대한 구체화 및 사용자 만족도와의 관계 관련 연구를 확인하여 이를 파악하고자 한다. 즉, 기존 문헌을 통해 편향을 반영한 추천 시스템 개발의 필요성을 파악해 보고자 한다. 이러한 연구 목적을 바탕으로 본 논문에서 설정한 연구 질문은 다음과 같다.
RQ1. 추천 시스템의 편향을 파악할 수 있는 평가 관점이 있는가?
RQ2. 추천 시스템의 편향과 사용자 만족도가 관련이 있는가?
RQ3. 편향 완화 및 사용자 만족도 향상을 위한 접근법은 무엇인가?
먼저, 추천 시스템의 편향은 평가 관점으로 정확도만 중시하는 구조에서 발생하였다. 즉, 추천 시스템을 평가하는 다른 관점을 파악함으로써 편향의 유무 및 정도를 확인할 수 있게 된다[8]. 두 번째로 편향과 사용자 만족도의 관계를 파악하여 편향이 추천 시스템에 미치는 직접적인 영향을 파악한다. 마지막으로, 기존 연구를 중심으로 편향 완화 및 사용자 만족도를 향상시키는 접근법을 정리해보고자 한다.
본 논문의 구성은 다음과 같다. 2장에서는 본 연구를 위한 연구 방법 및 문헌 검색 및 도출 과정을 설명한다. 3장에서는 연구 동향, 추천 시스템의 평가 관점 분석, 만족도와의 관계 분석 등의 연구 결과를 제시한다. 4장에서는 본 연구 질문에 대한 결과 요약 및 제언을 포함하며, 마지막으로 5장에서 본 연구의 요약, 기여점 및 한계점을 제시한다.
Ⅱ. 연구 방법
2-1 문헌 연구 고찰 방법
문헌고찰은 기존 문헌의 연구 결과들을 정리하는 것이며, 크게 서술적 고찰과 체계적 고찰로 구분된다. 서술적 고찰은 전문가가 가진 식견을 통해 기존 문헌들을 고찰하고 이를 저자가 주관적인 관점으로 종합하는 것이다. 따라서, 문헌을 선정하는 과정 및 종합 등의 방법이 저자별로 다르고 체계적이지 않다는 문제점이 있으며, 이로 인해 주관적 오류의 가능성이 있다[9]. 반면, 체계적 고찰은 특정 연구 문제 또는 주제 영역 등과 관련된 모든 식별 가능한 연구를 이용하여 해석한다[10]. 또한, 체계적인 고찰 단계를 구성하여 각각의 단계와 과정에 대한 신뢰수준이 높으며, 객관적이고 검증 가능한 방법을 사용하여 연구 주제에 대한 검증이 가능하다[11].
본 연구에서는 앞서 언급한 체계적 고찰 방법의 장점을 반영하기 위해, 다수의 연구에 활용된 방법인 Kitchenham(2004)의 문헌고찰 방법을 활용하였다.
2-2 연구 절차
본 연구에서 활용한 Kitchenham(2004)의 문헌고찰 문헌 검색 및 선택 절차 방법은 [표 1]에 나타나 있다.
검색 및 1차 선택, 2차 선택, 최종 선택 총 3단계로 구성된다. 검색 및 1차 선택 단계에서는 문헌 검색 및 추출을 위해 문헌고찰 프로토콜을 개발한다. 이후 개발한 프로토콜을 바탕으로 검색을 진행하고, 제목과 초록을 통해 1차 문헌을 선택한다. 본 연구에서 개발한 문헌고찰 프로토콜은 다음과 같다: (i) 추천 시스템의 알고리즘 관련 내용이 아닌, 이론적 내용을 담은 학술 논문일 것, (ii) 사용자 관점에 대해 논의한 학술 논문일 것, (iii) 학술 데이터베이스에서 원문을 확인할 수 있는 학술 논문일 것. 이후, 2차 선택 단계에서는 문헌의 질과 연구와의 연관성을 고려하여 전체적인 내용을 바탕으로 2차 문헌을 선택한다. 마지막으로, 최종 선택 단계에서는 2차 선택된 문헌을 최종적으로 검토하고, 최종 문헌을 확정한다. 이후 최종 문헌의 참고문헌을 검토하여, 이 중 문헌의 질 및 연구와의 연관성이 적합한 일부를 추가 선택한다.
2-3 연구 대상
구체적인 연구 문헌 선정 방법에 따른 연구 대상 도출은 [그림 1]을 통해서 진행되었다. 연구 대상을 선정하는 과정에서 데이터베이스의 키워드 검색, 연구자의 검토 및 선별, 참고문헌 파악, 최종 선정 문헌 확인 등의 과정이 포함되었다. 먼저, 문헌 검색을 위한 대상 기간은 2012년부터 2022년으로 하였으며, 해외 문헌 검색을 위해 ACM Digital Library와 국내 문헌 검색을 위해 DBpia 데이터베이스를 활용하였다. ACM Digital Library에서는 추천 시스템의 최고 권위 학회인 Recsys 게재를 추가 조건으로 두고, ‘bias’, ‘user satisfaction’, ‘filter bubble’, ‘algorithmic user bias’, ‘diversity’, ‘user centric’ 총 6가지 키워드를 포함 조건으로 검색하였다. DBpia에서는 ‘추천 시스템’과 더불어, ‘편향’, ‘필터 버블’ 2가지 키워드를 포함 조건으로 검색하였다.
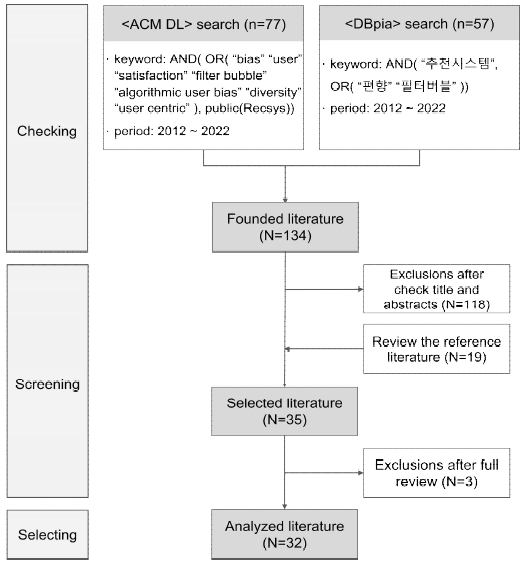
Procedure for selecting research literature*"This study used a couple of Korean keywords to search for domestic papers, and we included them as they are in this figure. “
본 조건을 통해 도출된 문헌이 총 134편이었으며, 이 중 제목과 초록 검토로 118편의 문헌을 제외하였다. 선택된 문헌들의 참고문헌 중 19편의 문헌을 추가로 선정하였고, 전체 선정 문헌 중 전문 검토로 3편의 문헌을 제외하였다. 이후 최종 분석 대상 문헌은 총 32편의 문헌을 선정하였다.
Ⅲ. 연구 결과
3-1 연구 현황 분석
추천 시스템의 편향 및 사용자 만족도 관련 최근 연구 현황 파악을 위해 연도별 연구 빈도수를 분석하였으며, 구체적인 결과는 [그림 2]와 같다.
연도별 연구 빈도수를 확인해본 결과, 전반적으로 상승하는 추세를 보였다. 2014년 이후부터 점차 편향의 문제점 및 사용자의 만족도 개선을 위한 연구가 진행되었음을 알 수 있으며, 그중 2021년이 가장 활발한 것을 알 수 있다.
선정된 연구 문헌들 상세는 [표 2]에 나타나있다. [표 2]에 제시된 것처럼, 본 논문 분석에 포함된 32편의 문헌은 R1부터 R32로 제시함으로써 가독성을 향상시켰으며, 이후부터는 코드를 통해 설명한다. 선정된 문헌에 대하여 저자들의 공동 상세 리뷰를 통해 해당 논문의 연구 주제를 추출하였고, 이에 대한 결과는 표에 자세히 나타나있다.
주요 연구 주제는 평가 관점, 사용자 만족도 향상, 사용자 특성에 따른 편향 수용도 및 추천, 인기 항목에 대한 편향(popularity bias), 필터 버블(filter bubble), 편향 및 발전 총 6가지로 나눌 수 있었다. [표 3]은 주요 연구 주제와 해당 문헌 및 빈도에 관해 설명한다. 이 중, 평가 관점 관련 문헌이 가장 빈도수가 높았고 그중 대부분은 다양성 향상을 위한 연구임을 확인하였다.
또한, 전체 문헌은 추천 시스템의 편향 완화를 위한 연구, 사용자의 관점에서 만족도 향상을 위한 연구 크게 두 가지 목적을 띈다. 편향 완화를 위한 연구에서는, 정확도를 넘어선 여러 평가 관점을 중점으로 논의하였다. 또한, 편향의 종류로는 인기 항목 편향(popularity bias)과 필터 버블(filter bubble) 등 사용자에 대한 편향 2가지로 나타났다.
사용자의 관점에서 만족도 향상을 위한 연구에서는, 사용자의 특성 분류 혹은 다양성 측면에 대해 논의하였다.
3-2 추천 시스템 평가 관점 분석
앞서 언급한 것처럼, 기존에는 추천 시스템의 평가를 위해 정확성만을 단일 관점으로 활용하였고, 이로 인해 편향 및 필터 버블이 발생하는 문제점이 있었다. 이러한 한계를 해결하기 위해, 본 연구에서는 [표 3] 내 Evaluation perspectives의 주제를 연구한 15편의 문헌을 상세 분석하여 총 네 가지의 추가 평가 관점을 개발하였다. [표 4]는 정확성을 포함한 총 5가지의 추천 시스템 평가 관점에 대해 제시한다.
정확성(Accuracy)은 추천 시스템이 사용자에게 추천한 아이템에 대한 실제 사용자의 평가를 얼마나 정확하게 예측하였는가에 관한 것이며, 실제 데이터와 추천된 결과 데이터를 비교함으로써 결과를 도출한다. 즉, 이는 추천 시스템의 단순한 성능을 평가하는 것이며, 추가로 Precision, Recall 등의 값도 활용될 수 있다.
다양성(Diversity)은 현재 추천 시스템이 사용자에게 추천한 아이템과 이전에 추천했던 아이템들 사이, 혹은 현재 추천한 아이템과 사용자의 기존 선호도에 따른 프로필 사이의 차이가 어느 정도인지에 관한 것이다[28]. 즉, 추천 시스템이 특정한 조건에만 치중되지 않고 다양한 아이템을 추천할 수 있는지의 능력을 평가하는 것이다.
롱테일(Long-tail)은 일반적인 추천에 많이 활용되는 인기 항목이 아닌 비인기 항목이 사용자에게 얼마나 많이 추천되었는가에 관한 것이다[17, 18]. 이는 소수의 사용자에게만 추천될 수 있는 소수의 비인기 항목을 얼마나 잘 추천할 수 있는가를 평가하는 것이다.
참신성(Novelty)과 우연성(Serendipity)은 모두 사용자에게 새로운 추천 항목을 제공한다는 점에서 공통점이 있다. 다만, 참신성은 기존 추천 결과 대비 얼마나 새로운 아이템을 추천할 수 있는지, 우연성은 사용자의 선호도 안에서의 예상치 못함을 중점으로 한다는 차이가 있다[28].
도출된 5가지의 평가 관점은 정확성을 제외한 다양성, 롱테일, 참신성, 우연성은 추천 시스템의 편향을 파악하는 데 활용될 수 있다. 예를 들어, 특정한 고객에게 추천을 제공하는 모델의 정확성이 높다 하더라도, 참신성과 우연성이 떨어진다면 해당 모델은 필터 버블의 문제를 겪고 있는 것으로 판단할 수 있다.
3-3 추천 시스템의 평가 관점 및 만족도 관계 분석
본 연구의 목적은 추천 시스템의 편향과 만족도 간 관계를 파악하는 것이다. 이를 위해, 앞서 3-2절에 도출된 추천 시스템의 평가 관점을 활용하여, 두 가지 방향의 분석을 진행하였다. 먼저, 기존 문헌의 상세 분석을 통해 평가 관점 간 관계성을 파악하였고, 이와 동시에 특정한 평가 관점 및 만족도 사이의 관계성을 파악하였다. 각각은 [표 5], [표 6]에 나타나 있다.
[표 5]에 나타나 있는 것처럼, 추천 시스템 내 다섯 가지 평가 관점 간 기존 연구 내 연관성을 확인할 수 있다. 예를 들어, R12 논문 내 발견되는 하나의 관계는 참신성이 다양성에 긍정적인 영향을 끼친다는 것이다. 또한, R20-2, R22-3은 특정한 조건(*) 하에서 발견되는 특징이다. 본 조건은 극단적인 Premise의 반영을 의미한다. 즉, 예를 들어 R20-2의 경우, 사용자에게 급격한 다양성을 고려한 추천은 정확도에 부정적인 영향을 끼친다는 것을 의미한다. 이 외에 다양한 관계들이 발견되며 주요한 관점 간 분석은 [그림 3]에 나타나 있다.
[표 6]은 추천 시스템 평가 관점과 사용자 만족도 간 관계를 나타낸다. 관점은 추천 시스템 내 다섯 가지 평가 관점을 의미하며, 사용자 만족도는 사용자 선호도 등을 포함한 일반적 의미를 뜻한다. [표 5]와 마찬가지로, R7-2, R12-1, R13-3, R13-4는 평가 관점의 정도가 극단적으로 올라갔을 때(*)에 따른 사용자 만족도와의 관계를 의미한다. 또한, R8-1, R29-1은 대체로 관계성을 갖는다는 것을 의미하며, 때때로는 반대로 작용한다는 의미이다.
본 추천 시스템 간 관계 및 만족도 간 관계를 요약한 결과는 [그림 3]과 같다. 이 중 우리는 세 가지의 주요한 관계성을 파악할 수 있다. 첫째, 참신성이 우연성에 영향을 주고, 우연성이 만족도에 직접적인 영향을 미친다[7, 8, 13, 22, 26, 27, 34, 41]. 둘째, 다양성이 만족도에 직접적인 영향을 미친다[12, 13, 20, 34, 38, 41]. 셋째, 정확도와 다양성은 반비례 관계이다[7, 13, 34]. 이와 더불어, 만족도에 긍정적 영향을 미치는 평가 관점은 정확성, 다양성, 참신성, 우연성 4가지가 있었다. 그 중, 정확성, 참신성, 우연성은 일정 수치 이상을 가지게 되면(***) 만족도가 감소하게 된다[6, 13, 22, 27, 40].
3-4 사용자의 특성 및 편향 관계 분석
추천 시스템의 평가 관점 간 관계, 사용자 만족도와의 관계와 더불어 연구 문헌 분석 내 추가적인 도출 결과는 추천 시스템이 사용자의 특성에 따라 차별적으로 작동될 수 있다는 것이다. 이와 관련하여, 사용자의 특성과 추천 시스템 평가 관점 및 사용자 만족도와의 관계를 분석하였고, 이는 [표 7]에 나타나 있다.
구체적으로, 사용자의 나이, 성별 등의 인구 통계학적 요소와 호기심, 개방성, 수용도 등의 여러 특성을 고려하였으며, 이 중 주요한 영향 관계로는 4가지를 확인할 수 있었다.
첫째, 사용자의 추천 아이템에 대한 수용도가 높아지면, 추천 아이템의 다양성이 향상한다. 이에 따라 사용자의 추천 아이템에 대한 만족도가 상승하는 효과가 있다[25].
둘째, 사용자의 나이가 많을수록 더 다양한 것에 더욱 만족하는 경향이 있다[16]. 셋째, 사용자의 호기심이 클수록 다양성, 우연성, 참신성의 효과가 좋다[6, 7, 16]. 넷째, 인기 항목 자체에 관한 관심이 적은 사용자일수록 인기 항목 편향이 미치는 영향이 크며, 이는 사용자의 만족도에 영향을 미친다[17, 18, 30].
Ⅳ. 논의 및 제언
4-1 연구 질문에 대한 논의
본 연구에서 설정한 세 가지 연구 질문에 대하여, 앞서 3장에서 제시한 문헌 분석 결과를 정리한 결과를 제공한다.
먼저, 첫 번째 연구 질문은 추천 시스템의 편향을 파악할 수 있는 평가 관점에 대한 유무이다. 본 연구에서 파악한 추천 시스템의 주요 평가 관점은 정확성, 다양성, 롱테일, 참신성, 우연성 총 5가지이다. 앞서 언급한 것처럼, 정확성은 추천 아이템의 정확도에 대한 것이며, 나머지 4개는 모두 사용자 관점에서 추천 아이템의 새로움 즉, 편향에 관한 것이다. 편향은 특정한 사용자 혹은 주제에 극도로 치중하는 것을 의미하며, 이에 따라 정확성이 매우 높은 상태가 된다. 또한, 편향은 다양성 및 롱테일을 감소시키며, 다양성 감소는 우연성 및 참신성을 감소시키는 경향이 있다. 즉, 전체 5개의 평가 관점 중 대체로 정확성과 다른 4개의 지표는 반비례 관계를 갖고, 이에 따라 편향을 파악할 수 있는 평가 관점으로 활용할 수 있다.
두 번째 연구 질문은 추천 시스템의 편향과 사용자 만족도 간 연관성에 대한 관계이다. 앞서 언급한 것처럼, 추천 시스템의 편향이 심해지면 사용자의 기존 선택들, 사용자 프로필에 유사한 것만 추천하는 필터 버블로 이어진다. 그렇게 되면, 정확성이 과도하게 높아지게 되고, 다양성이 매우 낮아지게 된다. 이에 따라 만족도가 감소한다. 따라서, 추천 아이템의 편향이 사용자의 만족도에 유의미한 영향을 미친다. 또한, 사용자의 특성에 따라 정도가 달라질 수 있다. 나이가 많을수록 추천 아이템의 다양성이 만족도에 미치는 영향이 크다. 또한, 호기심이 많을수록 우연히 취향에 적합한 아이템을 추천받았을 때 더 만족하는 우연성 효과가 크다.
마지막 연구 질문은 편향 완화 및 사용자 만족도 향상을 위한 접근법의 제시이다. 편향 완화를 위해 주요 5가지 평가 관점이 논의되었다. 이 중, 다양성 향상을 통한 만족도 향상의 방법이 가장 주로 논의되는 방향이다. 이는 참신성과 우연성과 관련이 있다. 참신성과 우연성은 다양성과 긍정적인 영향 관계이므로, 이 둘을 조절하여 적절한 수준의 다양성을 고려하는 것이 중요하다. 또한, 사용자의 만족도는 사용자의 특성에 따라 달라지는 경향이 있다. 수용도는 다양성에 긍정적인 영향을 미치며, 수용도가 높은 경우 만족도가 높다. 호기심은 다양성, 참신성, 우연성에 긍정적인 영향을 미치며, 인기 항목에 대한 관심도가 낮을수록 인기 항목 편향의 영향을 많이 받는다. 평가 관점이 만족도에 영향을 미치지만, 이는 사용자 개인이 가진 평가 관점별 선호도에 따라 그 정도는 달라질 수 있다는 것이다.
4-2 제언
추천 알고리즘이 발전함에 따라 편향의 문제점이 대두되고 있다. 추천 시스템의 편향은 크게 인기 항목 편향과 사용자 편향 두 가지로 나누어진다. 편향 완화를 위해 정확성 외의 다양성, 롱테일, 참신성, 우연성 총 4가지 추천 시스템의 평가 관점이 논의되었다. 이 중 정확성, 다양성, 우연성은 사용자의 만족도에 직접적 영향을 미치는 요소임을 확인했다. 편향이란 정확성이 매우 높은 것을 의미하며, 이는 반비례 관계인 다양성을 매우 낮은 수치로 이끈다. 이에 따라 만족도가 낮아지는 결론에 다다른다. 따라서 편향이 만족도에 유의미한 영향을 미치는 것을 알 수 있다.
또한, 이는 사용자의 특성에 따라 영향의 정도가 달라진다. 특성으로는 수용도, 나이, 호기심, 선호도 등이 있으며, 이를 고려하여 다양성의 정도를 조절함으로써 사용자별 더욱 효과적인 만족도 향상에 도움이 될 수 있다. 더 나아가, 편향 완화를 통한 만족도 향상을 위해 이와 관련한 방향성에 대한 고민이 필요하다. Exploration은 새로운 가치를 찾아내기 위해 기존과는 다른 새로운 추천을 하는 것으로, 이를 통해 다양성을 증가시킬 수 있다. 또한, Exploitation은 고객의 평가 결과 등의 데이터를 통해 학습한 결과를 추천하는 것이다. 추천 시스템의 편향을 완화하며 동시에 사용자의 만족도를 높이기 위해서는 Exploration과 Exploitation의 적절한 균형이 중요하다. 이는 MAB(Multi-Armed Bandit) 알고리즘[43]을 통해 가능한데, MAB을 활용하면 개별 사용자에게 맞는 균형을 정할 수 있고, 장기적으로 추천 알고리즘의 발전에 도움이 될 수 있다.
Ⅴ. 결 론
본 연구는 추천 시스템의 편향 및 만족도 관련 국내외 문헌을 통해 추천 시스템의 편향과 사용자 만족도의 관계, 편향 완화 및 사용자 만족도 향상을 위한 접근법을 분석하였다. 추천 시스템의 편향과 사용자의 만족도는 관련이 있으며, 편향이 만족도에 부정적인 영향을 준다. 사용자의 만족도는 편향의 정도에 따라 달라지며, 이는 모두 주관적인 요소로, 사용자 개개인의 특성에 따른다. 편향을 판단하는 평가 관점으로 정확성, 롱테일, 다양성, 참신성, 우연성 5가지가 있으며, 이들이 각각 편향 완화와 사용자의 만족도 향상에 영향을 준다. 정확성이 과도하게 높은 경우를 편향이라 말하며, 이는 롱테일, 다양성, 참신성, 우연성의 조절로 완화할 수 있다. 사용자의 만족도는 개인의 특성에 따라 다를 수 있으며, 다양성, 우연성의 조절로 향상할 수 있다.
국내 연구는 추천 시스템의 편향에 관한 연구가 미진하다. 또한, 편향과 사용자의 만족도를 함께 분석한 국내 문헌은 부재한 것으로 확인했다. 이에 따라, 본 연구는 국내외 추천 시스템의 편향과 사용자의 만족도 관련 결합 분석을 진행한 첫 문헌이라는 의의를 갖는다. 또한, 이후 본 연구가 사용자 만족도 향상과 관련하여 국내 추천 시스템의 발전에 도움이 될 수 있다.
그러나, 모든 학술 데이터베이스를 확인하지 못했으며, 데이터 수집이 앞서 이루어졌기 때문에 출판된 모든 문헌이 포함되지 않을 수 있다. 또한, 이론적인 방법을 제안하였으나, 실질적 알고리즘의 연계 방법은 제안하지 못했다는 한계를 가진다. 따라서, 연구의 객관성 등에 한계점이 있음을 밝힌다.
향후 연구에서는 다양한 시나리오에 대한 여러 수준의 다양성/우연성에 관한 연구가 진행되어야 할 것이다. 현재는 단일 수준에 관한 다양성의 변화 및 정도를 파악하는 것에 그치고 있다. 그러나, 추천 시스템에 대한 만족도는 사용자가 처한 상황에 따라 다를 수 있으므로, 개인화의 발전을 위해 다양한 시나리오를 적용한 다양성과 우연성의 여러 수준 비교 연구가 이루어져야 할 것이다. 또한, 사용자의 다양성 선호 정도에 따라 만족도를 최대화할 수 있는 최적의 추천 결과가 달라질 수 있다. 향후 연구에서는 사용자의 다양성 선호 정도를 분류하고, 이에 따른 더욱 발전된 개인화 수준의 추천에 관한 연구가 진행되어야 할 것이다.
Acknowledgments
본 연구는 과학기술정보통신부 및 정보통신기획평가원의 SW중심대학 지원사업(2017-0-00096), 한국연구재단의 기초연구사업(NRF-2021R1G1A1094019)의 연구결과로 수행되었음.
References
- Dong-Man Kim, Chul-Hyun Lee. "A Study on Systematic Review of Korean Literatures about Recommendation System based on Deep-Learning ." Journal of The Korean Association of Artificial Intelligence Education, Vol. 1, No. 1, pp.51-64, Mar. 2020, http://www.dbpia.co.kr/journal/articleDetail?nodeId=NODE10529513, .
-
J. B. Schafer, J. A. Konstan and J. Riedl, “E-Commerce Recommendation Applications”, Journal of Data Mining and Knowledge Discovery, Vol.5, No.1, pp.115-153, Jan. 2001
[https://doi.org/10.1023/A:1009804230409]
-
J. E. Son, S. B. Kim, H. J. Kim and S. Z. Cho, “Review and Analysis of Recommender Systems”, Journal of the Korean Institute of Industrial Engineers, Vol. 41, No. 2, pp. 185-208, Apr. 2015
[https://doi.org/10.7232/JKIIE.2015.41.2.185]
-
Datta, A., Tschantz, M. C., & Datta, A. “Automated experiments on ad privacy settings.” Proceedings on privacy enhancing technologies, Vol. 2015, No. 1, pp. 92-112, Mar. 2015
[https://doi.org/10.48550/arXiv.1408.6491]
-
Verma, Shikha. “Weapons of Math Destruction: How Big Data Increases Inequality and Threatens Democracy.” Vikalpa, vol. 44, no. 2, Jun. 2019, pp. 97–98
[https://doi.org/10.1177/0256090919853933]
-
Chen, Li, et al. "How serendipity improves user satisfaction with recommendations? a large-scale user evaluation." The world wide web conference, New York, NY, USA, pp. 240–250, May. 2019
[https://doi.org/10.1145/3308558.3313469]
- Hwang Yong Suk, et al. "An Introductory Study on Fairness Concept, Measuring Methods and Institutional Plan of Recommendation Algorithm : Focusing on Experts’ Awareness", Korean Journal of Broadcasting & Telecommunications Research, pp. 169-206, 2021.
-
Ekstrand, Michael D., et al. "User perception of differences in recommender algorithms." Proceedings of the 8th ACM Conference on Recommender systems, New York, NY, USA, pp. 161-168, Oct. 2014
[https://doi.org/10.1145/2645710.2645737]
-
Hyeong Sik Ahn and Hyun Jung Kim, "An introduction to systematic review" Journal of the Korean Medical Association, Vol. 57, No. 1, pp 49-59, Jan. 2014
[https://doi.org/10.5124/jkma.2014.57.1.49]
-
B. J. Kim, “Analysis of trends in Character Education research through a systematic review of the literature: Focusing on Network Text Analysis and Multidimensional Scaling”, Journal of Moral & Ethics Education, Vol. , No. 59, pp. 185-213, May. 2018
[https://doi.org/10.18338/kojmee.2018..59.185]
- B. Kitchenham, “Procedures for Performing Systematic Reviews”, Keele University, Technical Report TR/SE-0401, Jul. 2004.
-
Shi, Lei. "Trading-off among accuracy, similarity, diversity, and long-tail: a graph-based recommendation approach." Proceedings of the 7th ACM Conference on Recommender Systems, New York, NY, USA, pp. 57-64, Oct. 2013
[https://doi.org/10.1145/2507157.2507165]
-
Kaminskas, Marius, and Derek Bridge. "Diversity, serendipity, novelty, and coverage: a survey and empirical analysis of beyond-accuracy objectives in recommender systems." ACM Transactions on Interactive Intelligent Systems, New York, NY, USA, pp. 57-64, Mar. 2017
[https://doi.org/10.1145/2507157.2507165]
-
Bigdeli, Elnaz, and Zeinab Bahmani, "Comparing accuracy of cosine-based similarity and correlation-based similarity algorithms in tourism recommender systems.", IEEE International Conference on Management of Innovation and Technology, Bangkok, Thailand, pp. 469-474, Sep. 2008
[https://doi.org/10.1109/ICMIT.2008.4654410]
-
Kitchenham, Barbara, and Pearl Brereton. "A systematic review of systematic review process research in software engineering." Information and software technology, USA, pp. 2049–2075, Dec. 2013
[https://doi.org/10.1016/j.infsof.2013.07.010]
-
Wang, Ningxia, and Li Chen. "User Bias in Beyond-Accuracy Measurement of Recommendation Algorithms.", Fifteenth ACM Conference on Recommender Systems, New York, NY, USA, pp. 133-142, Sep. 2021
[https://doi.org/10.1145/3460231.3474244]
-
Abdollahpouri, Himan, et al. "The unfairness of popularity bias in recommendation.", Advances in Information Retrieval, Lisbon, Portugal, pp. 35–42, Apr. 2020
[https://doi.org/10.1007/978-3-030-45442-5_5]
-
Abdollahpouri, Himan, et al. "User-centered evaluation of popularity bias in recommender systems." Proceedings of the 29th ACM Conference on User Modeling, Adaptation and Personalization, New York, NY, USA, pp. 119-129, Mar. 2021
[https://doi.org/10.1145/3450613.3456821]
-
Calero Valdez, André, Martina Ziefle, and Katrien Verbert. "HCI for recommender systems: the past, the present and the future." Proceedings of the 10th ACM Conference on Recommender Systems, New York, NY, USA, pp. 123-126, Sep. 2016
[https://doi.org/10.1145/2959100.2959158]
-
Bhadani, Saumya. "Biases in Recommendation System." Fifteenth ACM Conference on Recommender Systems, New York, NY, USA, pp. 855-859, Sep. 2021
[https://doi.org/10.1145/3460231.3473897]
- In-sik Kim and JaMee Kim, "Youtube Algorithm and Confirmation Bias" Proceedings of the KACE, pp. 71-74, Jan. 2021, http://www.dbpia.co.kr/journal/articleDetail?nodeId=NODE10530792, .
-
Hwang Yong Suk and Kitae Kim, "The Survey of Recommendation System Research Trends and Methods", Journal of Cybercommunication Academic Society,ᅠVol. 36, No. 2, pp. 221-253, Jun. 2019
[https://doi.org/10.36494/JCAS.2019.06.36.2.221]
-
Jun, Junyong, et al., "A Verification about the Formation Process of Filter Bubble with Personalization Algorithm", Journal of Korea Multimedia Society, Vol. 21, no. 3, pp. 369-381, Mar. 2018
[https://doi.org/10.9717/KMMS.2018.21.3.369]
-
Kim Hyoeun, "Fairness Criteria and Mitigation of AI Bias", The Korean Psychological Association, vol. 40, no. 4, pp. 459-485, Dec. 2021
[https://doi.org/10.22257/kjp.2021.12.40.4.459]
-
Nguyen, Tien T., et al., "Exploring the filter bubble: the effect of using recommender systems on content diversity." Proceedings of the 23rd international conference on World wide web, New York, NY, USA, pp. 677-686, Apr. 2014
[https://doi.org/10.1145/2566486.2568012]
-
Jaewon Choi and Lee, Hong Joo, "An Integrated Perspective of User Evaluating Personalized Recommender Systems : Performance-Driven or User-Centric", The Journal of Society for e-Business Studies, vol. 17, no. 3, pp. 85-103, Aug. 2012
[https://doi.org/10.7838/jsebs.2012.17.3.085]
-
Matt, Christian, et al. "Escaping from the filter bubble? The effects of novelty and serendipity on users' evaluations of online recommendations.", Proceedings of the 2014 International Conference on Information Systems, Auckland, New Zealand, Dec. 2014
[https://doi.org/10.7892/BORIS.105398]
-
Wang, Shanfeng, et al. "Multi-objective optimization for long tail recommendation." Knowledge-Based Systems, Vol, 104, No. C, pp. 145-155, Jul. 2016
[https://doi.org/10.1016/j.knosys.2016.04.018]
-
Krishnan, Sanjay, et al. "A methodology for learning, analyzing, and mitigating social influence bias in recommender systems." Proceedings of the 8th ACM Conference on Recommender systems, New York, NY, USA, pp. 137-144, Oct. 2014
[https://doi.org/10.1145/2645710.2645740]
-
Abdollahpouri, Himan, Robin Burke, and Bamshad Mobasher. "Controlling popularity bias in learning-to-rank recommendation." Proceedings of the eleventh ACM conference on recommender systems, New York, NY, USA, pp. 42-46, Aug. 2017
[https://doi.org/10.1145/3109859.3109912]
-
Resnick, Paul, et al. "Bursting your (filter) bubble: strategies for promoting diverse exposure.", Proceedings of the 2013 conference on Computer supported cooperative work companion, New York, NY, USA, pp. 95-100, Feb. 2013
[https://doi.org/10.1145/2441955.2441981]
-
Tomlein, Matus, et al. "An Audit of Misinformation Filter Bubbles on YouTube: Bubble Bursting and Recent Behavior Changes." Fifteenth ACM Conference on Recommender Systems, New York, NY, USA, pp. 1-11, Mar. 2021
[https://doi.org/10.1145/3460231.3474241]
-
Park, Yoon-Joo, and Alexander Tuzhilin. "The long tail of recommender systems and how to leverage it." Proceedings of the 2008 ACM conference on Recommender systems, New York, NY, USA, pp. 11-18, Oct. 2008
[https://doi.org/10.1145/1454008.1454012]
-
Zhou, Tao, et al. "Solving the apparent diversity-accuracy dilemma of recommender systems." Proceedings of the National Academy of Sciences, Vol. 107. No. 10, pp. 4511-4515, Mar. 2010
[https://doi.org/10.1073/pnas.1000488107]
-
Tkalcic, Marko, and Li Chen. "Personality and recommender systems." Recommender systems handbook, pp. 715-739, 2015.
[https://doi.org/10.1007/978-1-4899-7637-6_21]
-
Yao, Yuan, and F. Maxwell Harper. "Judging similarity: a user-centric study of related item recommendations.", Proceedings of the 12th ACM Conference on Recommender Systems, New York, NY, USA, pp. 288-296, Sep. 2018
[https://doi.org/10.1145/3240323.3240351]
-
Ekstrand, Michael D., et al. "Letting users choose recommender algorithms: An experimental study." Proceedings of the 9th ACM Conference on Recommender Systems, New York, NY, USA, pp. 11-18, Sep. 2015
[https://doi.org/10.1145/2792838.2800195]
-
Ekstrand, Michael D., and Martijn C. Willemsen. "Behaviorism is not enough: better recommendations through listening to users.", Proceedings of the 10th ACM conference on recommender systems, New York, NY, USA, pp. 221-224, Sep. 2016
[https://doi.org/10.1145/2959100.2959179]
-
Nguyen, Tien T., et al. "User personality and user satisfaction with recommender systems.", Information Systems Frontiers, Vol. 20, No. 6, pp. 1173-1189, Sep. 2018
[https://doi.org/10.1007/s10796-017-9782-y]
-
Ziegler, Cai-Nicolas, et al. "Improving recommendation lists through topic diversification.", Proceedings of the 14th international conference on World Wide Web, New York, NY, USA, pp. 22-32, May 2005
[https://doi.org/10.1145/1060745.1060754]
-
Parapar, Javier, and Filip Radlinski. "Towards Unified Metrics for Accuracy and Diversity for Recommender Systems.", Fifteenth ACM Conference on Recommender Systems, New York, NY, USA, pp. 75-84, Sep. 2021
[https://doi.org/10.1145/3460231.3474234]
-
Harper, F. Maxwell, et al. "Putting users in control of their recommendations.", Proceedings of the 9th ACM Conference on Recommender Systems, New York, NY, USA, pp. 3-10, Sep. 2015
[https://doi.org/10.1145/2792838.2800179]
-
Sundar, Agnideven Palanisamy, et al. "Multi-armed-bandit-based shilling attack on collaborative filtering recommender systems.", 2020 IEEE 17th International Conference on Mobile Ad Hoc and Sensor Systems (MASS), Delhi, India, pp. 347-355, Dec. 2020.
[https://doi.org/10.1109/MASS50613.2020.00050]
저자소개
2019년~현 재: 광운대학교 정보융합학부 학사과정
※관심분야 : 데이터 사이언스, 추천 시스템, 프로세스 마이닝 등
2012년 : 경북대학교 컴퓨터학부 (공학사)
2019년 : 포항공과대학교 IT융합공학과 (공학박사)
2019년~2020년: 삼성SDS 책임연구원
2020년~현 재: 광운대학교 정보융합학부 조교수
※관심분야 : 인간-컴퓨터상호작용, 기계학습, 사용자 경험 등
2013년 : 울산과학기술원 테크노경영학부 (경영학사)
2018년 : 울산과학기술원 경영공학부 (공학박사)
2018년~2019년: 포항공과대학교 박사 후 연구원
2019년~2020년: 한국생산기술연구원 선임연구원
2021년~현 재: 광운대학교 정보융합학부 조교수
※관심분야 : 프로세스 마이닝, 자연어 처리, 산업 인공지능, 기계학습 등