
Emotion vs. Function: The Effects of AI Speaker’s Recognition on Product Attitude and Continuance Intention
Copyright ⓒ 2019 The Digital Contents Society
This is an Open Access article distributed under the terms of the Creative Commons Attribution Non-CommercialLicense(http://creativecommons.org/licenses/by-nc/3.0/) which permits unrestricted non-commercial use, distribution, and reproduction in any medium, provided the original work is properly cited.
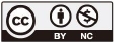
Abstract
Recently, It is important for AI device to figure out the emotions and then handle the issues in order to provide customized UX. For this purpose, we conducted a research to empirically analyze how AI speaker's emotional aspects(Emotional intelligence and social presence) vs. functional aspects(design typicality and product innovativeness) influenced on product attitude. In order to verify, we collected data from 250 users of AI speakers and utilized structural equation modeling(SEM). As a result, it turned out that the emotional intelligence and social presence of AI speaker had significantly positive effect on product attitude. Design typicality and product innovativeness were positively associated with product attitude. Additionally, Product attitude also had a positive effect on continuance intention.
초록
최근에는 AI device가 개인화된 경험을 제공할 수 있도록 감정을 파악하여 분석 및 처리하여 반응하는 것은 중요하다. 이를 위하여, 본 연구는 AI 스피커의 감성적 측면(감성지능과 사회적 실재감)과 기능적 측면(디자인 전형성과 제품 혁신성)이 제품태도에 어떠한 영향을 미치는지를 실증적으로 분석하였다. 이를 검증하기 위해 250명의 AI 스피커 사용자로부터 자료를 수집하고 구조방정식모형(SEM)을 사용하였다. 분석 결과, AI 스피커의 감성지능과 사회적 실재감은 제품태도에 유의한 긍정적 영향을 미치는 것으로 나타났다. 디자인전형성과 제품혁신성은 제품태도와 유의한 관계가 있는 것으로 나타났다. 또한 제품태도는 지속적 사용의도에 긍정적인 영향을 미치는 것으로 나타났다.
Keywords:
AI Speaker, Emotional Intelligence, Social Presence, Design Typicality, Product Innovativeness키워드:
AI 스피커, 감성지능, 사회적 실재감, 제품혁신성, 디자인전형성Ⅰ. Introduction
A college student, Jisoo, getting ready for college, asks her AI speaker “I haven’t got the jeans for my dating, I ordered it on Sunday. When will be my order shipped?” Most AI speaker are likely to answer, “Yes, They will be delivered in two days.” On the other hand, AI speaker with the emotional intelligence(EI) may answer “I am sorry. You must get disappointed that you've got it. It was delayed because of an issue of the delivery system. I will make it delivered in two days for you to wear the nice jeans to go out.” Likewise, the research result that AI can understand human's emotions better than humans has been recently published[1] but it serves just for the role to recognize and then assist and humans have been dominant in the domain where we should understand a context overall or we empathize and sympathize with others. The most optimized decision making of AI speaker is with interactivity. It needs to autonomously control the execution and predict and recognize what consumers need to do. It implies that AI needs to stick to the way of communication that includes customized and individualized services by interactively communicating with a user emotionally to figure out what the consumer really wants.
The recent issue is the emergence of AI that can communicate with a human and sympathize and recognize his/her emotions. There have been a lot of products so-called AI speakers and those have provided services not focused on its functions but on design and contents connected to it. However, voice recognition technology and AI technology are far different from each other. AI has been changing the way of interaction between humans and technology and creating disruptive momentum for growth in many ways. According to the survey firm in IT market, Gartner, AI speaker market is estimated to be worth 360 million dollars in 2015 and expected to be 3.6 billion in 2021. We can see that the market has been developing from a market for early adopters to a mass market. In addition, it predicts that integrated AI technologies, which can understand, analyze, and process human feelings and emotions to provide customized user experience(UX) adapted to any situations, will emerge as a result of the advancement of emotional AI systems[2]. According to the survey on AI speaker use state by consumer insight, however, one out of ten people uses AI speaker and it has mainly been used for selection of music, searching, and weather report[3]. Marketing science institute(MSI) chose the customer technology interface as one of the most important marketing themes to be studied from 2018 to 2020, which annually publishes research priority in the field of marketing research[4]. In particular, it pointed out that researches on the role of human-technology interface regarding marketing strategies like automation such as Alexa are imperative[4].
On the top of that, we lack preceding researches on consumers of AI speakers. Existing precedent researches focus on functions of AI speakers such as the accuracy of voice recognition technology and the measurement of efficacy. In short, most of researches have dealt with voice recognition design and technical trend based on document research on how precisely the device can recognize the voice orders of a human[5]. For these reasons, we conducted a research to practically investigate how using different types of devices can affect product attitude and continuance intention by comparing devices that apply the emotional aspects such as EI and social presence to functional aspects such as design typicality and product innovativeness, focusing not only on functional aspects but also on the importance of emotional aspects between AI speaker and a human.
Ⅱ. Conceptualizing the Hypotheses
2-1 AI Speaker
AI speaker can be defined as a personal assistant who performs an assigned task by understanding needs transferred in the form of voice and text of a person utilizing AI. AI speaker is a device equipped with intelligent personal assistant(IPA), and it provides information the user needs and helps his/her work by interacting with him/her[6]. For example, there are Alexa by Amazon, Siri by Apple, Google Assistant by Google, Bixby by Samsung. They provide a variety of services such as listening to music and searching for information based on IPA with voice recognition. IPA, embedded in devices such as speakers, smart phones, smart TVs, smart refrigerators, plays the roles described above. Devices equipped with IPA come in different forms and shapes and they have commonly been providing diversified services by communications among humans and things. These functions of IPA take charge of the roles of IoT hub or IoT gateway mentioned in many IoT researches and the roles are controlling the connection with other devices and managing data[7].
Those researches deal with devices assigned to play the roles and functional factors a system requires. One of those mentions that IoT gateway should be sensitive to changes in the environment, active to input and serve the user commands, located at a place with good accessibility, be functional to intuitively understand commands and directly answer with sound[8]-[9].
2-2 Emotional Intelligence and Product Attitude
EI means the ability to collect information of one’s own or others’ emotions and apply it so as to draw a positive outcome in a social situation[10]. EI is not the concept to explain an emotional state which can be experienced through social interaction of an individual but the concept to focus on individual capability to manage and control emotional experiences[11]. EI influences not only the sense of accomplishment related to personal career but also personal relationships corresponding to the satisfaction on one’s life[12]. In particular, he argues that it consists of two essential parts that divide into four sub-factors. Two essential parts are the domain of ability: emotional awareness and regulation and the domain of object: identification of oneself and others. Goleman draws four factors: emotional awareness of oneself/others and emotional regulation of oneself/others, by combining these domains. Among researches that conceptualize EI as scientific construct, there is a research that defines EI as a capability to monitor one’s and others’ emotions to differentiate them and use emotions to draw one’s thoughts and actions[13]. In addition, it is important for AI speaker to have the ability to understand emotions from interactions with consumers in the future to use it as interchange relations and form and maintain the relations with consumers emotionally[10]. Likewise, in order for AI speaker to cultivate and improve the relationship not only properly but effectively, it should maintain the ability to recognize emotions in any situations and interact with expressions of emotions; in this way, it can draw high levels of product attitude from consumers. Therefore, we suggest these hypothesis as below.
Hypothesis 1: AI speaker's emotional intelligence will be positively associated with product attitude.
2-3 Social Presence and Product Attitude
Social presence means psychological state in which a medium or a system used by a user is cognized as an actual social entity. Social presence means the state where a medium makes a user experience others being present by psychological cognizance[14]. Social presence can be called a psychological state where artificial or virtual social objects are experienced as present objects in sensory or non-sensory way; it develops when technology adopters assimilate with para-authentic of mediated objects or the artificial objects which is simulated and not an actual human[15]. Social presence has been defined depending on how media enable a user to have personal relations with other users[16]-[17]. Moreover, the importance of social presence in this online environment has been emphasized; Email, online bulletin boards, and SNS services based on IoT communication have been considered as a tool of social interaction and thus they have been emerging as something essential[18]. These preceding researches related to social presence provide room for discussion on what meaning social presence of a consumer has and how it functions in the online environment.
On the other hand, existing researches regarding AI and human-computer interaction(HCI), mention the importance of social interaction and social presence among users and devices and systems when it comes to computer agent systems or acceptance of AI devices and continuous usage of them; Furthermore, the importance of both a system formed with social interaction and social presence and a formation of relations between users is also mentioned[19]-[20]. Moreover, in the case of devices with IPA like AI speakers, social functions like conversations and communication as wells as traditional functions like searching for information, alarm, and shopping should be considered important[21]. Therefore, in the case of a device with AI technologies, the level of awareness on the device as a social object that can affect the continuous usage of the device should be considered as an important factor. The level of social presence can vary depending on delivery of stimulation of influential factors such as physical entity, nonverbal and social condition. In other words, social presence varies based on the level of physical entity, nonverbal and social condition communication media have[22]. Therefore, being based on interactivity theory, we built hypothesis on how a business facing reality can improve product attitude utilizing social presence.
Hypothesis 2: AI speaker's social presence will be positively associated with product attitude.
2-4 Design Typicality and Product Attitude
Typicality means how well an object can represent the category it belongs to and thus it can be defined as representativeness[23]. It is a concept related to the image or identity of a product consumers feel and experience. Saying that a thing has a high typicality means it represents the category well; It is not the concept made by manufacturers but consumers. In short, the concept is about how the public, consumers, are aware of the product. One argues that interpretation of information may vary depending on how information newly introduced to consumers correspond to the existing schema of them, and compatibility between the information and the category of the product affects the reviews on the product[24]. The reason why consumers prefer products with high typicality is that it easily comes across one’s mind and it has advantages when it comes to categorization of the object and accuracy; Faster learning speed for a product with high typicality can also be advantageous compared to the others[25]. However, there is a research that says high typicality can decrease the brand preference of consumers based on its survey on 500 brands[26]. Although these arguments on the relations between design typicality and product attitude are not compatible, this research is based on the argument that design typicality can be utilized as learning material to control product information and expression and can positively affect product attitude[27] and thus we draw hypothesis as below.
Hypothesis 3: AI speaker's design typicality will be positively associated with product attitude.
2-5 Product Innovativeness and Product Attitude
Product innovativeness means newness of innovation, a tendency to prefer something newer and edgier, when it is seen by cognitive level. Consumers recognize a product with actual changes in functions as something different from an existing image or identity. There have been many researches on product innovation since Thomson(1965) defined innovation is the process for an organization to create, accept, and implement a new line or product[28]. It was argued that when innovation is measured by the level of newness of a product, it can be divided into low, middle, high level of innovation and a product with high level of newness is highly innovative[29]. In addition, it was categorized innovation into two different types, where true innovation was discontinuous innovation and it means a product should be groundbreaking with a lot of changes including its usage and familiarity and thus it can be a totally different product[30].
As seen above, there are many concepts and types when it comes to product innovativness and they share the common goals: sustainable survival and growth of a business. After all, they find the common ground with the point that businesses struggle with the necessity of product innovation management to be dominant in the market. Based on existing precedent researches, we made hypotheses to examine how product innovativeness affects product attitude that consumers recognize focusing on high-tech AI speakers as our research objects.
Hypothesis 4: AI speaker's product innovativeness will be positively associated with product attitude.
2-6 Product Attitude and Continuance Intention
Continuance intention means the intention to use a specific system after trying it for the first time[31]. According to a research on continuance intention based on expectation-confirmation model, confirmation between pre/post usages affects satisfaction and usability about UX(user experience) and ultimately affects continuance intention[31].
Hypothesis 5: Product attitude will be positively related to continuance intention.
The research model are presented in Figure 1.
Ⅲ. Research Design
3-1 Method and Sample Characteristics
This research empirically analyzes the effects of emotional aspects(EI and social presence) recognize and functional aspects(product innovativeness and design typicality) perceived by AI speaker users on product attitude and continuance intention. Based on preceding research results, we verified credibility of research tool and modified questionnaires, and they were reviewed by AI specialists to be confirmed as the final questionnaires. This survey was done by the online panel of Embrain(www.embrain.com), an organization specialized in research. Data collection was conducted from November 1 to 14, 2019 and data from 250 people was used for analysis. Data analysis was done using SPSS 24.0 and AMOS 24.0 and we verified the hypotheses with structural equation modeling(SEM).
Personal characteristics of respondents are as seen in Table 1.
3-2 Measures
In order to measure EI in this research, we modified 16 questions of WLEIS(Wong and Law Emotional Intelligence Scale), developed by Wong and Law in 2002[32], which has been used in different researches[33]. WLEIS consists of 4 sub-factors: other’s emotion appraisal(OEA), use of emotion(UOE), self-emotion appraisal(SEA), and regulation of emotion(ROE); each has 4 questions and we used average values from measurement. Defining social presence as feelings to take a part in communication with AI speaker through social and psychological factors, we restructured and utilized the criteria of social presence developed by Park and Cho(2015)[34] based on the research of Burgoon and Hale(1987)[35]. There are examples of questions as follows: It seems that AI speaker is interested in communicating with me. AI speaker wants to have more conversations. AI speaker care about why I like it. AI speaker wants to build a closer relation with me communicating. AI speaker is eager to have a conversation with me.
In order to measure the level of typicality, we used questions for measurement modified and used by Choi, Park, and Kim(2001)[36] with a reference to the research by Hampton and Gardiner (1983)[37]. There are questions regarding the extent of typicality of AI speaker one uses and the extent to which the user considers AI speaker as the representative. With product innovativeness conceptualized as the extent to which one accepts newness of a product, using it, we modified the criteria used by Andrews and Smith(1996)[38], Sethi et al.(2001)[39] to write four questions. Product attitude was measured by four questions: I like the product. I prefer the product. I accept it positively. based on the research by Pan and Schmitt(1996)[40]. We defined continuance intention as the extent to which a user is willing to act to be provided with services at the expense of monetary value. Measurement items were used applying the intention for a user to keep using a specific system after trying it for the first time dealt with in the research of Bhattacherjee(2001)[31]; for example, I will keep using the AI speaker. I will stick with it and not buy a new one.
Ⅳ. Analyses
4-1 Reliability and Validation of Measuring Instruments
SPSS 24.0 was used for descriptive statistics and correlation analysis, and AMOS 24.0 was used for the hypothesis test because the structural equation modeling(SEM) would be suitable. The descriptive and correlation coefficients of the variables included in the study are shown in Table 1. In particular, there was a significant correlation between EI and social presence(r = .744, p <.001), product innovativeness and product attitude(r = .689, p <.001). As a result of checking the multicollinearity, it was determined that all the variables had a VIF(variation inflation factor) lower than 2.1 and did not affect the analysis results.
In accordance with the two-step approach to the SEM, confirmatory factor analysis was performed to verify the measurement model before verifying structural model. The goodness-of-fit index of the measurement model was acceptable for χ² = 351.117, df = 237, CFI = .975, TLI = .971, RMR = .035, RMSEA = .044 (CFI, TFI> .90; RMR <. 05; RMSEA <.05)[41]. The standardized factor loading of each measurement variable was generally more than .60, and the average variance extracted(AVE) of potential variables was higher than .50 and composit reliability(CR) was higher than .70. appeared[42]. Also, one item were used in the analysis by deleting one item of the continuous intention variable that showed low item-to-total with the standardized factor loading.
4-2 Hypotheses Analysis
The model fit of the hypothesized structural model was appropriately acceptable(χ² = 773.746, df = 247, CFI = .883, TLI = .870, RMSEA = .093). As presented in Table 4, The results of testing the hypothesis are as follows. First, EI acceptability was found to have a significant positive relationship with product attitude. Hypothesis 1 was supported(standardized path coefficient = .095, t = 2.249, p <.05). there was a significant positive relationship social presence and product attitude. Hypothesis 2 was supported(standardized path coefficient = .379, t = 7.412, p <.001). Second, design typicality was positively associated with product attitude(standardized path coefficient = .170, t = 2.255, p <.05). Product Innovativeness had a positive relationship with product attitude(standardized path coefficient = .435, t = 9.189, p <.001). Thus, hypotheses 3 and 4 were supported. Additionally, product attitude was positively associated with continuance intention(standardized path coefficient = .768, t = 10.826, p <.001).
Ⅴ. Discussion
The criteria of AI process assessment built in this research may guide practitioners on how to design and implement AI programs and be used to check if AI performs efficiently. This research can contribute to vitalizing AI-related researches as it discovers the essence of approach to AI speaker and methodically analyzes the formation of intention to use AI speaker. In this trend where interest and usage of AI speakers have been increasing, to predict the different responses by classifying emotional/functional aspects of AI speaker provides insight on AI technology development and strategic direction using AI speakers. Therefore, we expect that standards or norms in detail necessary for developing a product that meets the technical condition and the level of extent of AI speaker which can maximize the effect of communication can be built based on the research result. The results of this study are summarized as follows.
First, self-emotion appraisal, which is in one of the main domains, means the ability to recognize and express one’s own emotions. As the level of capacity to utilize emotions is higher, which enables one to regulate and control his emotions, mutual relationship will be more positively affected. As seen above, AI speaker with high EI adequately controls both his and others’ emotions so that it can meet the needs of the consumer as a compassionate device with flexibility. The argument that recognition by AI speaker users is closely related to usage motivation of them concurs with preceding research[43]. It implies that two-way communication with consumers is important and it should directly understand and respond to them. Based on the research results above, it is confirmed that EI and social presence are fundamental to build personal relations and to adapt to the society and they are also essential when it comes to customer satisfaction management that prioritizes the relations with customers. In addition, it is expected that AI speaker and a consumer can have emotional communication more easily as they have more similarity to one another. Recognition technology is able to show a certain result by performing by itself but sophisticated functions have been needed in the recent condition deluged by a lot of products with complicated services. Businesses, empathetic with consumers not in the case of supply-oriented system but a demand-oriented system and on-demand services, also need to provide customized solutions by integrating and analyzing necessary information. After all, not only innovative design and technology but insight on consumer needs are necessary to be successful in the field of AI; We need to focus on the emotional communication for UX-oriented products to perform better[44].
Second, when a certain product is more typical than reserved typical cases, the distortion of archetype decreases and we can notice that memory recall of familiar products positively affects product attitude of consumers. Preceding researches on product innovativeness too much focused on the nature of product innovation with strategic perspective to draw coherent results. This research, however, looked into how consumers accept product innovation with consumer-oriented perspective. As seen in the research result, consumers positively respond to technical complexity and the lack of familiarity from innovativeness of a product and they have more positive attitudes as the extent of newness is stronger.
In this context, this research may contribute to vitalize technical development and usages of emotional and functional rules in the field of AI adapting to the smart device environment where emotions matter. This research, making diagnosis of problems of leading technologies regarding AI speakers, suggests the directions of research and technology development to be combined with diversified industries in the future. Further researches are expected to investigate detailed data and cause-and-effect relationship by analyzing users’ responses to AI speakers with blogs, Youtube, and SNSs and the differences of relative recognition of consumers.
Acknowledgments
This work was supported by Hanshin University Research Grant.
References
- Kskao, 2018, The Future with AI [Internet]. Available: https://brunch.co.kr/@kakao-it/314
- Gartner, 2017, Artificial Intelligence, Immersive Experiences, Digital Twins, Event-thinking and Continuous Adaptive Security Create a Foundation for the Next Generation of Digital Business Models and Ecosystems [Internet]. Available: https://www.gartner.com/smarterwithgartner/gartner-top-10-strategic-technology-trends-for-2018/
- Consumer Insight, 2017, Hot AI Speaker Market, Cold Consumer Assessment [Internet]. Available: https://www.consumerinsight.co.kr/voc_view.aspx?no=2924&id=ins02_list&PageNo=1&schFlag=0
- Marketing Science Institute, 2018-2020 Research Priorities: Marketers’ Strategic Imperatives [Internet]. Available: https://www.msi.org/articles/marketers-top-challenges-2018-2020-research-priorities/
- G. E. Jo and S. I. Kim, “A study on User Experience of Artificial Intelligence speaker,” Journal of the Korea Convergence Society, Vol. 9, No. 8, pp. 127-133, 2018.
- K. Kabir, Smart Speakers-everything You Need to Know, What Hi-Fi? [Internet]. Available: https://www.whathifi.com/advice/smart-speakers-everything-you-need-to-know, (Accessed February 14, 2018).
-
S. Andreev, O. Galinina, A. Pyattaev, M. Gerasimenko, T. Tirronen, J. Torsner and Y. Koucheryavy, “Understanding the IoT Connectivity Landscape: A Contemporary M2M Radio Technology Roadmap, IEEE Communications Magazine, Vol. 53, No. 9, pp. 32-40, 2015.
[https://doi.org/10.1109/MCOM.2015.7263370]
-
A. R. Al-Ali, I. A. Zualkernan, M. Rashid, R. Gupta and M. Alikarar, “A Smart Home Energy Management System Using IoT and Big Data Analytics Approach, IEEE Transactions on Consumer Electronics, Vol. 63, No. 4, pp. 426-434, 2017.
[https://doi.org/10.1109/TCE.2017.015014]
-
D. F. Santos, H. O. Almeida and A. Perkusich, A Personal Connected Health System for the Internet of Things Based on the Constrained Application Protocol,” Computers & Electrical Engineering, Vol. 44, pp. 122-136, 2015.
[https://doi.org/10.1016/j.compeleceng.2015.02.020]
-
B. D. Kidwell, M. Hardesty, B. R. Murtha and S. B. Sheng, Emotional Intelligence in Marketing Exchanges,” Journal of Marketing, Vol. 75, No. 1, pp. 78-95, 2011.
[https://doi.org/10.1509/jm.75.1.78]
-
J. D. Mayer, P. Salovey and D. R. Caruso, Emotional Intelligence: New Ability or Eclectic Traits?,” American Psychologist, Vol. 63, No. 6, pp. 503-517, 2008.
[https://doi.org/10.1037/0003-066X.63.6.503]
- D. Goleman, Emotional intelligence, New York: Bantam Books, 1995.
-
P. Salovey and J. D. Mayer, “Emotional Intelligence. Imagination,” Cognition, and Personality, Vol. 9, pp. 185-211, 1990.
[https://doi.org/10.2190/DUGG-P24E-52WK-6CDG]
-
J. Fulk, C. W. Steinfield, J. Schmitz and J. G. Power, “A Social Information Processing Model of Media Use in Organizations,” Communication research, Vol. 14, No. 5, pp. 529-552, 1987.
[https://doi.org/10.1177/009365087014005005]
-
K. M. Lee, “Presence, Explicated,” Communication Theory, Vol. 14, pp. 27–50, 2004.
[https://doi.org/10.1111/j.1468-2885.2004.tb00302.x]
-
N. Kumar and I. Benbasat, “The Influence of Recommendations and Consumer Reviews on Evaluations of Websites,” Information Systems Research, Vol. 17, No. 4, pp. 425-439, 2006.
[https://doi.org/10.1287/isre.1060.0107]
-
W. Wang and I. Benbasat, “Recommendation Agents for Electronic Commerce: Effects of Explanation Facilities on Trusting Beliefs,” Journal of Management Information Systems, Vol. 23, No. 4, pp. 217-246, 2007.
[https://doi.org/10.2753/MIS0742-1222230410]
-
B. D. Homer, L. P. Jan and B. Linda, “The Effects of Video on Cognitive Load and Social Presence in Multimedia-learning,” Computers in Human Behavior, Vol. 24, No. 3, pp. 786-797, 2008.
[https://doi.org/10.1016/j.chb.2007.02.009]
-
N. Lee and O. Kwon, “Para-social Relationships and Continuous Use of Mobile Devices,” International Journal of Mobile Communications, Vol. 11, No. 5, pp. 465-484, 2013.
[https://doi.org/10.1504/IJMC.2013.056956]
-
M. Heerink, B. Kröse, V. Evers and B. Wielinga, “Assessing Acceptance of Assistive Social Agent Technology by Older Adults: the Almere Model,” International Journal of Social Robotics, Vol. 2, No. 4, pp. 361-375, 2010.
[https://doi.org/10.1007/s12369-010-0068-5]
- H. J. Lee, “A Ghost in the Shell? Influences of AI Features on Product Evaluations of Smart Speakers with Customer Reviews,” Journal of Information Technology Services, Vol. 17, No. 2, pp. 191-205, 2018.
- J. Short, W. Ederyn and C. Bruce, The Social Psychology of Telecommunications, John Wiley & Sons, 1976.
-
J. Barney, “Firm Resources and Sustained Competitive Advantage,” Journal of Management, Vol. 17, No. 1, pp. 99-120, 1991.
[https://doi.org/10.1177/014920639101700108]
- Mandler, G. The Structure of Value: Accounting for Taste. in Affect and Cognition: The 17th Annual Carnegie Symposium, M. S. Clark and S. A. fiske(Eds.), Lawrence Erlbaum Associates, pp. 3-66, 1982.
-
C. B. Mervis and E. Rosch, “Categorization of Natural Objects,” Annual Review of Psychology, Vol. 32, pp.89-115, 1981.
[https://doi.org/10.1146/annurev.ps.32.020181.000513]
-
G. S. Carpenter and K. Nakamoto, “Consumer Preference Formation and Pioneering Advantage,” Journal of Marketing Research, Vol. 26, pp. 285-298, Aug, 1989.
[https://doi.org/10.1177/002224378902600303]
-
P. W. Henderson and J. A. Cote, “Guidelines for Selecting or Modifying Logos,” Journal of Marketing, Vol. 62, No. 2 pp. 14-30, 1998.
[https://doi.org/10.1177/002224299806200202]
-
V. A. Thompson, “Bureaucracy and Innovation,” Administrative Science Quarterly, pp. 1-20, 1965.
[https://doi.org/10.2307/2391646]
-
L. Lawton and A. Parasuraman, “The Impact of the Marketing Concept on New Product Planning,” Journal of Marketing, Vol. 44, pp. 19-25, Winter, 1980.
[https://doi.org/10.1177/002224298004400103]
-
P. W. Meyers and F. G. Tucker, “Defining Roles for Logistics During Routine and Radical Technological Innovation,” Journal of the Academy of Marketing Science, Vol. 17, No. 1, pp. 73-82, 1989.
[https://doi.org/10.1007/BF02726356]
-
A. Bhattacherjee, “Understanding Information Systems Continuance: An Expectation-confirmation Model,” MIS quarterly, pp. 351-370, 2001.
[https://doi.org/10.2307/3250921]
-
C. Wong and K. S. Law, “The Effects of Leader and Follower Emotional Intelligence on Performance and Attitude: An Exploratory Study,” The Leadership Quarterly, Vol. 13, pp. 243-274, 2002.
[https://doi.org/10.1016/S1048-9843(02)00099-1]
-
K. S. Law, C. Wong and L. J. Song, “The Construct and Criterion Validity of Emotional Intelligence and Its Potential Utility for Management Studies,” Journal of Applied Psychology, Vol. 89, No. 3, pp. 483–496, 2004.
[https://doi.org/10.1037/0021-9010.89.3.483]
-
K. I. Park and C. H. Cho, “Developing the Scale of Brand Social Presence : Focusing on Facebook,” The Korean Journal of Advertising, Vol. 26, No. 5, pp. 213-241, 2015.
[https://doi.org/10.14377/KJA.2015.7.15.213]
-
J. K. Burgoon and J. L. Hale, “Validation and Measurement of The Fundamental Themes of Relational Communication,” Communications Monographs, Vol. 54, No. 1, pp. 19-41, 1987.
[https://doi.org/10.1080/03637758709390214]
- I. H. Choi, J. Y. Park and E. J. Kim, “Effects of Design Prototypicality on the Evaluation of Product Design,” Journal of Consumer Studies, Vol. 12, No. 3, pp. 147-169, 2001.
-
J. Hampton and Gardiner, “Measure of Internal Category Structure: A Correlational Analysis of Normative Date,” British Journal of Psychology, Vol. 74, No. 4, pp. 491-516, 1983.
[https://doi.org/10.1111/j.2044-8295.1983.tb01882.x]
-
J. Andrew and D. C. Smith, “In Search of The Marketing Imagination: Factors Affecting the Creativity of Marketing Programs for Mature Products,” Journal of Marketing Research, Vol. 33, pp. 174-187, May, 1996.
[https://doi.org/10.1177/002224379603300205]
-
R. Sethi, D. C. Smith and C. W. Park, “Cross-functional Product Development Teams, Creativity, and the Innovativeness of New Consumer Products,” Journal of Marketing Research, Vol. 38,pp. 73-85, Feb, 2001.
[https://doi.org/10.1509/jmkr.38.1.73.18833]
-
Y. Pan and B. Schmitt, “Language and Brand Attitudes: Impact of Script and Sound Matching in Chinese and English,” Journal of Consumer Psychology, Vol. 5, No. 3, pp. 263-277, 1996.
[https://doi.org/10.1207/s15327663jcp0503_03]
-
J. C. Anderson and D. W. Gerbing, “Structural Equation Modeling in Practice: A Review and Recommended Two-step Approach,” Psychological Bulletin, Vol. 103, No. 3, pp. 411-423, 1988.
[https://doi.org/10.1037/0033-2909.103.3.411]
-
L. Hu and P. M. Bentler, “Cutoff Criteria for Fit Indexes in Covariance Structure Analysis: Conventional Criteria Versus New Alternatives,” Structural Equation Modeling: A Multidiscipinary Journal, Vol. 6, No. 1, pp. 1-55, 1999.
[https://doi.org/10.1080/10705519909540118]
-
C. Wong and K. S. Law, “The Effects of Leader and Follower Emotional Intelligence on Performance and Attitude: An Exploratory Study,” The Leadership Quarterly, Vol. 13, pp. 243-274, 2002.
[https://doi.org/10.1016/S1048-9843(02)00099-1]
-
J. Y. Chung and M. J. Kim, “A Study on Personalized Music Recommendation Model through Analysis on Users' Music Preference Factors,” Journal of Digital Contents Society, Vol. 19, No. 11, pp. 2041-2047, Nov, 2018.
[https://doi.org/10.9728/dcs.2018.19.11.2041]
2006년 : 경희대학교 국제경영학부 (경영학사)
2008년 : 경희대학교 기술경영학과 (경영학석사-MIS)
2013년 : 경희대학교 국제경영학과 (경영학박사-MIS)
2014년~2017년: 성결대학교 파이데이아학부 조교수
2017년~현 재: 한신대학교 IT경영학과 조교수
※관심분야: 빅데이터(Big Data), 인공지능(AI), 텍스트마이닝(Text-Mining), IoT
2001년 : 서울시립대학교 경제학부 (경제학사)
2003년 : 서울시립대학교 경영학과 (경영학석사)
2010년 : 서울시립대학교 경영학과 (경영학박사-마케팅)
2014년~현 재: 성결대학교 파이데이아학부 조교수
※관심분야: B2B 마케팅(B2B Marketing), 감성지능(Emotional Intelligence), 감정노동(Emotional Labor)