
A study on lane path contents for car navigation systems to resolve road inconvenience
Copyright ⓒ 2019 The Digital Contents Society
This is an Open Access article distributed under the terms of the Creative Commons Attribution Non-CommercialLicense(http://creativecommons.org/licenses/by-nc/3.0/) which permits unrestricted non-commercial use, distribution, and reproduction in any medium, provided the original work is properly cited.
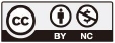
Abstract
As shown in many recent studies, the different traffic flow characteristics between lanes are very important in terms of generating and managing traffic information. If avoiding congested road sections is an important purpose of navigation systems, avoiding congested lanes by using traffic state information by lane will also be an important function of navigation systems for resolving road inconvenience in the future. Therefore, this paper proposes a lane-path calculation method required for lane-path services which will be a core content of navigation systems in the future. For this purpose, we proposed a lane-path calculation method for uninterrupted roads by observing the traffic state by each lane. This study collected the travel time by each lane on the Gangbyeon Expressway(Gangbyeonbuk-ro) and analyzed the difference in travel time between lanes. In addition, this study built a road network of the road section and proposed a shortest lane-path calculation method using this, and as a result of comparing the shortest and longest lane-path, there was a significant effect on the reduction of travel time according to the optimal choices of lanes when driving in uninterrupted roads.
초록
최근 많은 연구에 의해 밝혀진 바와 같이 차로 간 교통류 특성의 상이함은 교통정보를 생성 및 관리하는 측면에서 매우 중요하다. 혼잡한 도로구간을 회피하는 것이 내비게이션의 중요한 목적이라면 차로별 교통상황 정보를 활용하여 혼잡한 차로를 회피하는 것 또한 미래의 도로이용 불편 해소를 위한 내비게이션의 중요한 기능일 것이다. 이에 본 연구에서는 미래 내비게이션의 핵심 콘텐츠인 차로경로 서비스를 위해 반드시 요구되는 차로경로 계산 방법을 제안하였다. 이를 위해 차로별 교통상황을 관측하고, 이를 이용하여 연속류 도로에서의 차로경로(lane-path) 계산 방법을 제안하였다. 강변북로를 대상으로 차로 통행시간을 수집하였고 차로 간 통행시간 차이를 분석하였다. 또한 도로구간을 차로도로망의 구축과 이를 활용한 최단차로경로 계산 방법을 제시하였고, 이를 통해 최단차로경로와 최장차로경로를 비교한 결과, 연속류 도로 주행 시 차로의 적절한 선택에 따라 통행시간 단축 효과가 큰 것으로 나타났다.
Keywords:
Car Navigation System, Lane Path, Lane-by-lane Traffic State, Probe Vehicle, Shortest Lane Path키워드:
차량용 내비게이션, 차로경로, 차로별 교통상태, 프로브차량, 최단거리차로경로Ⅰ. Introduction
The world has evolved rapidly with the emergence of the Internet at the end of the 20th century, and this is accelerating with the rise of smartphones, IoT, and big data. In the field of road traffic, various technologies that were possible only to imagine in the past have been realized or will be realized in the near future, and technologies such as smartphone-based road services, autonomous driving technologies using precision electronic maps, and lane level location reference technologies are already being actively studied and developed [1]-[3].
The provision of traffic information through VMS (Variable Message Sign), which gained much attention as a breakthrough technology when the term ATIS (Advanced Traveller Information Systems) first appeared, is now regarded as a technology of the past. Now, there are many apps available that can access traffic information through a single touch on a smartphone. The traffic information provided in the early days of ATIS was produced by applying models to a small amount of data to estimate the current situation. However, with the rise of mobile devices such as smartphones, it became much easier to acquire large amounts of data on the move than before, which enabled the provision of traffic information based on big data.
While the quality of the traffic information is gradually improving with the advance of technology, road link-based information still dominates the traffic information market. Traffic information (speed, travel time, congestion, etc.) has long been expressed by one of the many attributes of a link, based on the network of roads consisting of links and nodes. This is a method of dividing the road based on a specific facility on the road, defining each divided section as a link, and managing the traffic information by the static and dynamic properties of the corresponding link, and is based on the assumption that the traffic situation in all lanes on the road are homogeneous, without considering the number of lanes of the road.
A lane is, however, a carriageway with the right-of-way that is separated by the lane marking on the same road section, and has different traffic flow characteristics (speed, traffic volume, traffic density) depending on the lane. The complexity of the road network rapidly increases if we recognize the lane as an independent object, so the road has been simplified for use, assumed as a single object (link) with homogeneous characteristics. As a result, we are not able to connect with actual research while agreeing with the need for research to observe the myriad interactions between lanes and utilize the results.
Many studies on the analysis of traffic flow characteristics by lane have been performed consistently by researchers from other countries. [4] reviewed the analysis for micro-simulation calculations, and discovered that the traffic flow parameters of the simulation model should be applied differently for each lane, and that the same uniform factors cannot be applied to all lanes. [5] is one of the earliest studies to analyze the traffic flow by each lane from a behavioral perspective. The study classified the drivers into aggressive and non-aggressive drivers, and assumed that aggressive drivers would use the passing lane while non-aggressive drivers use the outer lanes because aggressive drivers overtake non-aggressive drivers in free traffic flows. In congested traffic flow conditions, the study assumed that the speed difference between cars decreased and that aggressive drivers are redirected from the overtaking lane to other lanes, and demonstrated this by experiments. [6] showed that traffic flow characteristics by each lane were different by presenting an analysis index of traffic volume ratio by lane, and [7] also discovered that the distribution of traffic volume by lane had a particular form. In Korea, [8] developed a methodology for estimating travel time by lane using a V2X-based traffic information system. [9] analyzed the traffic flow characteristics by lane in uninterrupted roads with the concept of lane-wise speed reversal, and as a result, found that lane-wise speed reversal occurred preeminently when congestions occur on uninterrupted roads.
The fact that the traffic characteristics are different for each lane within the same road section is very important in terms of generating and managing traffic information. For example, when only one lane is congested in the same 4-lane road section, knowing the information makes a huge difference in the efficiency of road use. Therefore, it is necessary to improve the precision of ATIS information through reflecting these characteristics and to ultimately develop lane-path contents for car navigation systems that reflect this. For this purpose, this study proposes a lane-path calculation method for uninterrupted roads by observing the traffic state in each lane, where a lane-path is defined as a route that includes the lane use information on a path from the origin to the destination by considering the lanes on a multi-lane road as mutually independent objects.
Ⅱ. Development of lane-path contents
2.1. Traffic state observation method by lane
The traffic information of each lane can be analyzed through the point data by each lane acquired through an inductive loop detector, which has advantages such as ease of data acquisition and plenty of research outputs on relevant traffic flow theories. However, it is difficult to observe the detailed change of traffic flow caused by the continuity of road alignment and the friction due to changing lanes. In addition, it is difficult for point-based speed data to provide information on lane-by-lane travel time. The lane-by-lane travel time can be defined as the travel time of a vehicle using only one lane from the beginning to the end of a road section, but the speed observed at a point makes it difficult to represent the travel speed by lane since many vehicles change lanes while driving. [5] argues that the most important reason for this lane change behavior is to maintain the driver’s desire speed, which in turn causes separation by lane in non-congested states. [8] argued that if the drivers could recognize the travel time by each lane, they would have the valuable opportunity to choose the lane that minimizes travel time when passing through an area where an incident has occurred .
Therefore, there is a need for techniques to observe the changes in traffic flow by each lane for the entire road section. In order to satisfy this demand, we can divide the road section into very short intervals and install detectors at every point, but this is not a possible and/or realistic alternative due to a high cost.
Another alternative is to use probe vehicles. This refers to using probe vehicles to perform a microscopic method of detecting the change in the traffic flow of each lane while driving along the target road section according to the average traffic flow. For this purpose, the probe vehicle should be able to synchronize the trajectory, images, and events during driving, and playback the information after driving, and identify the location of each event and the situation at that time. We may use MMS (Mobile Mapping System) vehicles that collect road environment data in real-time while driving [10], but this is difficult in reality because MMS vehicles are very expensive. Therefore, this study used a lane-specific portable traffic information observation device which is easy to install on the probe vehicle to observe the travel time by lane on uninterrupted roads and to analyze the lane-path characteristics.
2.2. Collection & analysis of traffic state data by lane
This study used the Lane-by-lane Traffic Observing System (LTOS) based on a mobile device that is easy to attach to vehicles to observe the travel time by lane [11]. The LTOS is a device that was developed as a mobile device app to observe traffic information by lane and operates on iOS-based devices with GPS capability. The main functions include tracing vehicle trajectories, storing the driving screens, and reproducing the stored trajectory and driving information. In addition, it provides functions such as vehicle speed display, heading, event storage/playback, location (coordinate) display on maps, and the current time. Fig. 1 shows the main screen of the LTOS.
When a probe vehicle equipped with LTOS drives in one lane, the vehicle locations are stored every second, along with a video of the front of the vehicle. At this time, the stored coordinates, i.e., the trajectory of the vehicle, is temporally synchronized with the video. The stored trajectory is used to calculate the vehicle speeds, changes in deceleration speed, and location tracking, while the driving video is used to monitor the traffic state according to the location and time of the corresponding trajectory.
The LTOS provides the user with an event key function which allows to store specific events at every desired point. For example, when an accident is witnessed, it can be used to store the accident point, or to accurately store the merging or diverging areas. The event information can also be viewed through a list, and the location, time, and video of the corresponding point can be viewed by selecting the desired information.
In this study, we observed the travel times of the median (the first lane in terms of Korean lane numbering convention) lane and center lane (the second lane in terms of Korean lane numbering convention) on the Gangbyeon Expressway using two vehicles. For the eastbound direction of the Gangbyeon Expressway, Sungsan Bridge was set as the starting point and Dongho Bridge as the end point, and the observation started at 14:30. The corresponding road section is suitable for analyzing the change in traffic according to the entry and exit of vehicles because there are entry and exit ramps connected to the first lane in most of the connecting bridges. The analysis was performed with the assumption that the first lane is affected by entry and exit and the second lane is relatively less affected.
There are 10 bridges in the target section, and after dividing the entire target road (Gangbyeon Expressway) into 9 sections based on each bridge, we calculated the travel time, distance, and speed of each section. Fig. 2-a and 2-b show the travel time (tt1i, tt2i) and speed (υ1i, υ2i) of the median and center lane by sections, respectively. The space mean speed of the median lane was 55.9kph and 58.9kph for the Center lane, and in terms of the travel speed by section, the Center lane was higher in all sections except for the 2nd, 8th, and 9th sections. These results are considered to be due to the fact that the majority of the ramps that enter and exit the target road (east of Gangbyeon Expressway) are installed connected to the first lane. The Median lane is concentrated with vehicles that change lanes to use the entry ramp connected to the first lane and vehicles approaching the main lane through the entry ramp, so the congestion increases because weaving occurs at the same time.
The median and center lanes show different results when comparing the travel distances (d1i, d2i) between each lane. On curved roads, since the radius curvature of the inner and outer lanes are different, the travel distance of the outer lane is usually longer. The travel distance of the vehicle on the Median lane was about 13.633km, and the distance of the Center lane was about 13.747km, showing a difference of 114m, but the difference in travel time was considered to be insignificant.
In sections 6 and 7 (Hangang Bridge~Banpo Bridge), the travel time between the lanes showed the biggest difference (25%), which was due to the large volume of traffic entering from the northern end of Hangang Bridge to the Gangbyeon Expressway, which in turn led to a severe bottleneck in the median lane. Strategic traffic management methods are required to reduce the difference in traffic speed between lanes at times with heavy entering and exiting traffic, and inducing the use of outer lanes in advance by guiding lane use may one of these methods [11].
2.3. Lane-path calculation method development
This section observed the change in travel cost according to the choice of lanes by analyzing the lane-path. The lane-path includes the lane selection information within the path that connects the origin to the destination by considering the lanes on a multi-lane road as mutually independent objects. This requires a new approach to a road network modeling because it is difficult to use conventional road network expression methods that do not consider the lanes.
In general, studies on geographic information systems use the link-node centerline method which uses the center line of a road to express a road network. This method assumes that the link expressing a road section represents the corresponding road section, and that the traffic flow characteristics of all the lanes in the corresponding road section are the same in terms of traffic. However, as mentioned in the introduction, many studies have proved that the traffic flow characteristics by lane are different, and because of these differences, the choice of path, travel time, driving safety, and convenience are different, so a more detailed method of expressing the road network is required than the existing methods for better traffic services, especially to improve the service of next-generation car navigation systems.
Since roads are composed of one or more lanes, a road section can be laterally divided by the number of lanes, which again can be longitudinally divided. Therefore, one road section can be expressed by a single lane of a certain length, or by a combination of lane cells (Fig. 3-b). In other words, the lane cell is a concept of dividing a road into regular intervals based on a lane. A road composed of one or more lanes consists of at least as many lane cells as the number of lanes. The number of lane cells increase or decrease when additionally reflecting the discontinuity of lanes due to the physical increase/decrease of lanes, suspension, construction, and accidents.
One lane cell has the same physical driving environment. In other words, we assume that every vehicle traveling in the same lane cell never experiences a change in the travel speed or acceleration/deceleration due to physical changes in the road. Further, we assume that no lane change occurs from the lane cell perspective. Although this is different from the actual driving environment where vehicles change lanes at any location on the road (unless they violate the lane change regulations), this is a reasonable assumption considering the fact that lane changes do not occur frequently when driving on a typical road. Due to the nature of the lane cell, we can ensure homogeneous traffic flow characteristics within a single lane cell and maintain independence between the lane cells.
The lane cell can be expressed as a link with inherent road traffic characteristics, and referred to as a lane-link to distinguish it from the link in the conventional link-node centerline method (Fig. 3-c). Since a lane has directional characteristics, a lane-link is also only possible for one-way traffic.
The movement between adjacent lane cells takes place at the contact point, which is called a lane-node. The lane-node serves as a connection to the lane-link and is also a point for changing lanes. A lane change is made through a lane-changing-link which connects a lane-node of one lane to another of an adjacent lane at the same location. The lane change is completed as the driver makes the decision and moves from the previous lane to the target lane in response to the surrounding driving environment, and so the travel cost according to this behavior can be calculated by the time difference from the point of the driver’s decision to the point of driving in the target lane when considering only the time, but it may also be considered by distinguishing from the driver’s decision to the driving time in the previous lane and the driving time in the target lane. This study adopted the latter method and set the travel cost of the lane change link to 0. In other words, we assumed that there is no additional cost other than the driving time according to changing lanes.
Through introducing this concept, one road section is represented by a lane-network composed of a lane-link and lane-node, and the start and end nodes are processed by introducing a virtual super-node and cost-free links to connect them. The lane selection at the start node can be determined by considering the lane of the previous link or the rotation at the intersection, but this discussion is left out because the target road in this study is a section of an uninterrupted road.
This study used a general algorithm for finding the shortest path to calculate the shortest lane-path in the road network. The Dijkstra algorithm [12] is a typical tree building algorithm that finds the shortest path across all of the road networks with non-negative link costs. So, it is possible to calculate the shortest lane-path by using the Dijkstra algorithm in the road network. However, since only two lanes are actually considered in the case of the target road, we can calculate the shortest path by comparing the travel time of each lane cell on the same location in the median and center lanes, which can be calculated using Equation (1) below.
(1) |
where N : total number of sections.
In general, a real road network consists of multiple road networks, and since there are many roads with 3 or more lanes, it is difficult to calculate the shortest lane-path by only a simple comparison of each lane cell within a road network as in the target road. Therefore, a general algorithm such as the Dijkstra algorithm is required to calculate the shortest lane-path between the origin and the destination.
This study analyzed the shortest lane-path using a single lane-path and Equation (1). As a result, the travel time in the first lane-path (only through the median lane) was 982 seconds and the second lane-path (only through the center lane) was 907 seconds, showing a difference of 7.6%. The travel time in the shortest lane-path calculated through Equation (1) was 886 seconds (travel distance: 13.7km), which used the first lane in the second, eighth, and ninth sections and the second lane for other sections. When using the first lane at the starting point, the vehicle changes lanes 2 times to follow the shortest lane-path. If you use the shortest lane-path, you can travel 9.8% faster than using only the median lane, and 2.3% faster than using only the center lane. On the other hand, the longest lane-path that takes the longest time is when you use the second lane in the second, eighth, and ninth sections and the first lane for other sections, which takes 1,003 seconds (travel distance: 13.6km) and 13.2% more than the travel time of the shortest lane-path.
While driving on a multi-lane road, the driver changes lanes several times because of the desire to maintain the desire speed or the selection of the next road, but since there is no problem of selecting roads in the target road section, changing lanes is only possible according to the desire to maintain the desire speed. A rational driver will move to a faster lane, and the result of selecting the optimal location to change lanes will be the shortest lane-path. On the contrary, the worst selection will result in the longest lane-path. The target road section is about 13km, and the difference in travel time between the shortest and longest lane-paths is 117 seconds. Assuming that the vehicle travels at 58.9kph, which is the average speed in the second lane, the difference is about 1.9km compared to the vehicle that travels by the longest lane-path. As such, the optimal choice of lanes makes a significant contribution to reducing travel time because there is a huge difference according to the choice of lanes when driving in an uninterrupted road.
Ⅳ. Conclusion
This study proposed a lane-path calculation method required for lane-path services, which is a core content of car navigation systems to resolve road inconvenience. For this purpose, we used a probe vehicle equipped with LTOS, a traffic state observation system, to observe the traffic flow characteristics of each lane while driving on the road according to the average traffic flow. This study collected travel time by each lane on the Gangbyeon Expressway, where the travel time on the median lane which connects with multiple entry and exit ramps was slightly higher than that of the center lane, and the difference in travel time between these two lanes tends to increase with increased congestion. In addition, this study built a road network divided into multiple lane cells and proposed a method for calculating the shortest lane-path using this, and as a result of comparing the shortest lane-path with the longest lane-path, the optimal choice of lanes had a significant effect on reducing the travel time when driving in an uninterrupted road.
This study collected and analyzed data on only two lanes in an uninterrupted road, and further study should be conducted on more lanes as well as on the lane-path calculation algorithm performance in the future. In addition, research should also be carried out on interrupted roads as they have different traffic flow characteristics compared to uninterrupted roads.
References
-
Jeon, W-H., Yang, I., “Development of a location-based smartphone app reporting road problems with user participation”, Journal of Digital Contents Society, 19(10), p1825-1832, (2018).
[https://doi.org/10.9728/dcs.2018.19.10.1825]
-
Yang, I., Jeon, W-H., “Development of lane-level location data exchange framework based on high-precision digital map”, Journal of Digital Contents Society, 19(8), p1617-1623, (2018).
[https://doi.org/10.9728/dcs.2018.19.8.1617]
-
Yang, I., Jeon, W. H., Lee, H. M., “A Study on Dynamic Map Data Provision System for Automated Vehicle”, Journal of Korea Institute of Intelligent Transport System, 16(6), p208-218, (2017).
[https://doi.org/10.12815/kits.2017.16.6.208]
-
Carter, M., Rakha, H., Aerde, M.V., “Variability of traffic flow measure across freeway lanes”, Canadian Journal of Civil Engineering, 26(3), p270-281, (1999).
[https://doi.org/10.1139/l98-065]
-
Daganzo, C.F., “A behavioral theory of multi-lane traffic flow, Part I: Long homogeneous freeway sections”, Transportation Research Part B, 36, p131-158, (2002).
[https://doi.org/10.1016/s0191-2615(00)00042-4]
-
Amin, M.R., Banks, J.H., “Variation in freeway lane use patterns with volume, time of day, and location”, Transportation Research Record: Journal of the Transportation Research Board, 1934, p132-139, (2005).
[https://doi.org/10.1177/0361198105193400114]
-
Duret, A., Ahn, S., Buisson, C., “Lane flow distribution on a three-lane freeway: General features and the effects of traffic controls”, Transportation Research Part C: Emerging Technologies, 24, p157-167, (2012).
[https://doi.org/10.1016/j.trc.2012.02.009]
-
Rim, H., Oh, C., Kang, K., Kim, S., “Estimation of Lane-Level Travel Times in Vehicle-to-Vehicle and Vehicle-to-Infrastructure-Based Traffic Information System”, Transportation Research Record: Journal of the Transportation Research Board, 2243, p9-16, (2011).
[https://doi.org/10.3141/2243-02]
-
Yang, I., Jeon, W-H, Ki, S., Yoon, J., “Lane-wise travel speed characteristics analysis in uninterrupted flow considering lane-wise speed reversal”, Journal of Korea Institute of Intelligent Transport Systems, 15(5), p116-126, (2016).
[https://doi.org/10.12815/kits.2016.15.6.116]
-
Park, J-H, Yun, D-G, Sung, J-G, LEE, J-S, “The Measurement of Road Alignment Using GPS-IMU System”, Journal of Korean Society of Transportation, 30(5), p67-75, (2012).
[https://doi.org/10.7470/jkst.2012.30.5.061]
- Korea Institute of Civil Engineering and Building Technology (KICT), “Development of road network analysis technology based on road lanes (Phase I)”, Technical Report, KICT, (2013).
-
Dijkstra, E., “A note on two problems in connection with graphs”, Neuriche Mathematics, 1, p269-271, (1959).
[https://doi.org/10.1007/bf01386390]
저자소개
2011년: Ph.D. in Civil Engineering at Univ. of California, Irvine
2000년: 연세대학교 도시공학 석사
1998년: 연세대학교 도시공학 학사
2011년~현 재: 한국건설기술연구원 수석연구원
※관심분야: 첨단교통, 자율주행, C-ITS, 도로안전, 도로시설
2016년: 서울대학교 도시계획학 박사
2001년: 한양대학교 교통공학 석사
1999년: 한양대학교 교통공학 학사
2001년~현 재: 한국건설기술연구원 수석연구원
※관심분야: 도로안전, 무동력 교통수단, 모바일 앱, 도로시설